Open Access
ARTICLE
Multi-Scale Network for Thoracic Organs Segmentation
1 Department of Computer Science, Bahria University, Islamabad, Pakistan
2 Department of Information systems, College of Computer and Information Sciences, Jouf University, KSA
3 School of Computer Science and Engineering (SCE), Taylor’s University, Malaysia
4 Center for Smart Society 5.0 (CSS5), Faculty of Innovation and Technology (FIT), Taylor’s University, Malaysia
5 Department of Information Technology, College of Computers and Information Technology, Taif University, Taif, 21944, Saudi Arabia
* Corresponding Author: N.Z Jhanjhi. Email:
Computers, Materials & Continua 2022, 70(2), 3251-3265. https://doi.org/10.32604/cmc.2022.020561
Received 29 May 2021; Accepted 01 July 2021; Issue published 27 September 2021
Abstract
Medical Imaging Segmentation is an essential technique for modern medical applications. It is the foundation of many aspects of clinical diagnosis, oncology, and computer-integrated surgical intervention. Although significant successes have been achieved in the segmentation of medical images, DL (deep learning) approaches. Manual delineation of OARs (organs at risk) is vastly dominant but it is prone to errors given the complex irregularities in shape, low texture diversity between tissues and adjacent blood area, patient-wide location of organisms, and weak soft tissue contrast across adjacent organs in CT images. Till now several models have been implemented on multi organs segmentation but not caters to the problem of imbalanced classes some organs have relatively small pixels as compared to others. To segment OARs in thoracic CT images, we proposed the model based on the encoder-decoder approach using transfer learning with the efficientnetB7 DL model. We have built a fully connected CNN (Convolutional Neural network) having 5 layers of encoding and 5 layers of decoding with efficientnetB7 specifically to tackle imbalance class pixels in an accurate way for the segmentation of OARs. Proposed methodology achieves 0.93405 IOU score, 0.95138 F1 score and class-wise dice score for esophagus 0.92466, trachea 0.94257, heart 0.95038, aorta 0.9351 and background 0.99891. The results showed that our proposed framework can be segmented organs accurately.Keywords
Cite This Article
Citations
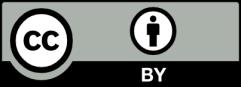