Open Access
ARTICLE
Machine Learning Based Depression, Anxiety, and Stress Predictive Model During COVID-19 Crisis
1 Department of Computer Science, King Khalid University, Muhayel Aseer, Saudi Arabia
2 Faculty of Computer and IT, Sana’a University, Sana’a, Yemen
3 Department of Information Systems, College of Computer and Information Sciences, Princess Nourah bint Abdulrahman University, Saudi Arabia
4 Department of Computer and Self Development, Preparatory Year Deanship, Prince Sattam bin Abdulaziz University, AlKharj, Saudi Arabia
5 Department of Natural and Applied Sciences, College of Community-Aflaj, Prince Sattam bin Abdulaziz University, Saudi Arabia
6 Computer Science Department, King Khaled University, KSA
7 Faculty of Science, Mathematics and Computer Science Department, Menoufia University, Egypt
* Corresponding Author: Fahd N. Al-Wesabi. Email:
Computers, Materials & Continua 2022, 70(3), 5803-5820. https://doi.org/10.32604/cmc.2022.021195
Received 25 June 2021; Accepted 26 July 2021; Issue published 11 October 2021
Abstract
Corona Virus Disease-2019 (COVID-19) was reported at first in Wuhan city, China by December 2019. World Health Organization (WHO) declared COVID-19 as a pandemic i.e., global health crisis on March 11, 2020. The outbreak of COVID-19 pandemic and subsequent lockdowns to curb the spread, not only affected the economic status of a number of countries, but it also resulted in increased levels of Depression, Anxiety, and Stress (DAS) among people. Therefore, there is a need exists to comprehend the relationship among psycho-social factors in a country that is hypothetically affected by high levels of stress and fear; with tremendously-limiting measures of social distancing and lockdown in force; and with high rates of new cases and mortalities. With this motivation, the current study aims at investigating the DAS levels among college students during COVID-19 lockdown since they are identified as a highly-susceptible population. The current study proposes to develop Intelligent Feature Subset Selection with Machine Learning-based DAS predictive (IFSSML-DAS) model. The presented IFSSML-DAS model involves data preprocessing, Feature Subset Selection (FSS), classification, and parameter tuning. Besides, IFSSML-DAS model uses Group Gray Wolf Optimization based FSS (GGWO-FSS) technique to reduce the curse of dimensionality. In addition, Beetle Swarm Optimization based Least Square Support Vector Machine (BSO-LSSVM) model is also employed for classification in which the weight and bias parameters of the LSSVM model are optimally adjusted using BSO algorithm. The performance of the proposed IFSSML-DAS model was tested using a benchmark DASS-21 dataset and the results were investigated under different measures. The outcome of the study suggests the development of specialized programs to handle DAS among population so as to overcome COVID-19 crisis.Keywords
Cite This Article
Citations
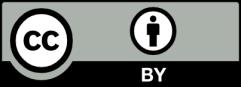