Open Access
ARTICLE
Disturbance Evaluation in Power System Based on Machine Learning
1 Department of Electrical Engineering, College of Engineering, Jouf University, Sakaka, 72388, Al-Jouf, Saudi Arabia
2 Department of Electrical Engineering, College of Engineering, Prince Sattam bin Abdulaziz University, Wadi Addawaser, 11991, Saudi Arabia
3 Department of Electrical Engineering, Aswan Faculty of Engineering, Aswan University, Aswan, 81542, Egypt
4 Department of Electrical Engineering, University of Engineering and Technology Peshawar, Pakistan
* Corresponding Author: Emad M. Ahmed. Email:
Computers, Materials & Continua 2022, 71(1), 231-254. https://doi.org/10.32604/cmc.2022.022005
Received 23 July 2021; Accepted 24 August 2021; Issue published 03 November 2021
Abstract
The operation complexity of the distribution system increases as a large number of distributed generators (DG) and electric vehicles were introduced, resulting in higher demands for fast online reactive power optimization. In a power system, the characteristic selection criteria for power quality disturbance classification are not universal. The classification effect and efficiency needs to be improved, as does the generalization potential. In order to categorize the quality in the power signal disturbance, this paper proposes a multi-layer severe learning computer auto-encoder to optimize the input weights and extract the characteristics of electric power quality disturbances. Then, a multi-label classification algorithm based on rating is proposed to understand the relationship between the labels and identify the various power quality disturbances. The two algorithms are combined to construct a multi-label classification model based on a multi-level extreme learning machine, and the optimal network structure of the multi-level extreme learning machine as well as the optimal multi-label classification threshold are developed. The proposed method can be used to classify the single and compound power quality disturbances with improved classification effect, reliability, robustness, and anti-noise performance, according to the experimental results. The hamming loss obtained by the proposed algorithm is about 0.17 whereas ML-RBF, SVM and ML-KNN schemes have 0.28, 0.23 and 0.22 respectively at a noise intensity of 20 dB. The average precision obtained by the proposed algorithm 0.85 whereas the ML-RBF, SVM and ML-KNN schemes indicates 0.7, 0.77 and 0.78 respectively.Keywords
Cite This Article
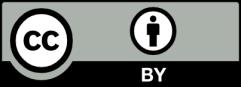