Open Access
ARTICLE
Deep Learning Control for Autonomous Robot
1 College of Engineering, Muzahimiyah Branch, King Saud University, Riyadh, 11451, Saudi Arabia
2 Laboratory for Analysis, Conception and Control of Systems, LR-11-ES20, Department of Electrical Engineering National Engineering School of Tunis, Tunis El Manar University, 1002, Tunisia
3 King Abdulaziz City for Science and Technology, Saudi Arabia
4 College of Computing and Information Technology, University of Bisha, Bisha, 67714, Saudi Arabia
* Corresponding Author: Saad Alhuwaimel. Email:
Computers, Materials & Continua 2022, 72(2), 2811-2824. https://doi.org/10.32604/cmc.2022.020259
Received 17 May 2021; Accepted 13 December 2021; Issue published 29 March 2022
Abstract
Several applications of machine learning and artificial intelligence, have acquired importance and come to the fore as a result of recent advances and improvements in these approaches. Autonomous cars are one such application. This is expected to have a significant and revolutionary influence on society. Integration with smart cities, new infrastructure and urban planning with sophisticated cyber-security are some of the current ramifications of self-driving automobiles. The autonomous automobile, often known as self-driving systems or driverless vehicles, is a vehicle that can perceive its surroundings and navigate predetermined routes without human involvement. Cars are on the verge of evolving into autonomous robots, thanks to significant breakthroughs in artificial intelligence and related technologies, and this will have a wide range of socio-economic implications. However, in order for these automobiles to become a reality, they must be endowed with the perception and cognition necessary to deal with high-pressure real-life events and make proper judgments and take appropriate action. The majority of self-driving car technologies are based on computer systems that automate vehicle control parts. From forward-collision warning and antilock brakes to lane-keeping and adaptive drive control, to fully automated driving, these technological components have a wide range of capabilities. A self-driving car combines a wide range of sensors, actuators, and cameras. Recent researches on computer vision and deep learning are used to control autonomous driving systems. For self-driving automobiles, lane-keeping is crucial. This study presents a deep learning approach to obtain the proper steering angle to maintain the robot in the lane. We propose an advanced control for a self-driving robot by using two controllers simultaneously. Convolutional neural networks (CNNs) are employed, to predict the car’ and a proportional-integral-derivative (PID) controller is designed for speed and steering control. This study uses a Raspberry PI based camera to control the robot car.Keywords
Cite This Article
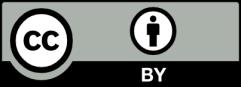