Open Access
ARTICLE
A Hybrid System for Customer Churn Prediction and Retention Analysis via Supervised Learning
1 Department of Computer Science, COMSATS University, Islamabad, Attock Campus, Pakistan
2 Department of Computer Science, COMSATS University, Islamabad, Islamabad Campus, Pakistan
* Corresponding Author: Khalid Iqbal. Email:
Computers, Materials & Continua 2022, 72(3), 4283-4301. https://doi.org/10.32604/cmc.2022.025442
Received 24 November 2021; Accepted 11 February 2022; Issue published 21 April 2022
Abstract
Telecom industry relies on churn prediction models to retain their customers. These prediction models help in precise and right time recognition of future switching by a group of customers to other service providers. Retention not only contributes to the profit of an organization, but it is also important for upholding a position in the competitive market. In the past, numerous churn prediction models have been proposed, but the current models have a number of flaws that prevent them from being used in real-world large-scale telecom datasets. These schemes, fail to incorporate frequently changing requirements. Data sparsity, noisy data, and the imbalanced nature of the dataset are the other main challenges for an accurate prediction. In this paper, we propose a hybrid model, name as “A Hybrid System for Customer Churn Prediction and Retention Analysis via Supervised Learning (HCPRs)” that used Synthetic Minority Over-Sampling Technique (SMOTE) and Particle Swarm Optimization (PSO) to address the issue of imbalance class data and feature selection. Data cleaning and normalization has been done on big Orange dataset contains 15000 features along with 50000 entities. Substantial experiments are performed to test and validate the model on Random Forest (RF), Linear Regression (LR), Naïve Bayes (NB) and XG-Boost. Results show that the proposed model when used with XGBoost classifier, has greater Accuracy Under Curve (AUC) of 98% as compared with other methods.Keywords
Cite This Article
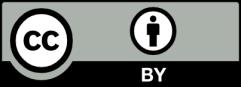