Open Access
ARTICLE
Week Ahead Electricity Power and Price Forecasting Using Improved DenseNet-121 Method
1 Electrical Engineering Department, College of Engineering, Najran University Saudi Arabia, Najran, 61441, Saudi Arabia
2 Department of Computer Science, COMSATS University Islamabad, Wah Campus, 22060, Pakistan
3 Faculty of Maritime Studies, King Abdulaziz University, Jeddah, 21589, Saudi Arabia
4 Department of Computer Science, Federal Urdu University of Arts, Science and Technology, Islamabad, 44000, Pakistan
5 School of Electrical Engineering and Computer Science, National University of Science and Technology, Islamabad, 44000, Pakistan
6 Department of Computer Science and Engineering, University of Engineering and Technology, Narowal Campus, Lahore, 54000, Pakistan
7 Department of Electrical Engineering, HITEC University, Taxila, 47080, Pakistan
8 College of Computer Science and Information Systems, Najran University, Najran, 61441, Saudi Arabia
* Corresponding Author: Ali Raza. Email:
Computers, Materials & Continua 2022, 72(3), 4249-4265. https://doi.org/10.32604/cmc.2022.025863
Received 07 December 2021; Accepted 09 February 2022; Issue published 21 April 2022
Abstract
In the Smart Grid (SG) residential environment, consumers change their power consumption routine according to the price and incentives announced by the utility, which causes the prices to deviate from the initial pattern. Thereby, electricity demand and price forecasting play a significant role and can help in terms of reliability and sustainability. Due to the massive amount of data, big data analytics for forecasting becomes a hot topic in the SG domain. In this paper, the changing and non-linearity of consumer consumption pattern complex data is taken as input. To minimize the computational cost and complexity of the data, the average of the feature engineering approaches includes: Recursive Feature Eliminator (RFE), Extreme Gradient Boosting (XGboost), Random Forest (RF), and are upgraded to extract the most relevant and significant features. To this end, we have proposed the DensetNet-121 network and Support Vector Machine (SVM) ensemble with Aquila Optimizer (AO) to ensure adaptability and handle the complexity of data in the classification. Further, the AO method helps to tune the parameters of DensNet (121 layers) and SVM, which achieves less training loss, computational time, minimized overfitting problems and more training/test accuracy. Performance evaluation metrics and statistical analysis validate the proposed model results are better than the benchmark schemes. Our proposed method has achieved a minimal value of the Mean Average Percentage Error (MAPE) rate i.e., 8% by DenseNet-AO and 6% by SVM-AO and the maximum accurateness rate of 92% and 95%, respectively.Keywords
Cite This Article
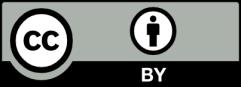