Open Access
ARTICLE
Breast Cancer Detection in Saudi Arabian Women Using Hybrid Machine Learning on Mammographic Images
1 Division of Radiology, Department of Medicine, Medical College, Najran University, Najran, 61441, Kingdom of Saudi Arabia
2 Department of Computer Science, COMSATS University Islamabad, Sahiwal Campus, Sahiwal, 57000, Pakistan
3 Department of Radiology, College of Medicine, Qassim University, Buraidah, 52571, Kingdom of Saudi Arabia
4 Department of Radiology, King Fahad Specialist Hospital, Buraydah, 52571, Kingdom of Saudi Arabia
5 Electrical Engineering Department, College of Engineering, Najran University, Najran, 61441, Kingdom of Saudi Arabia
6 Radiology Department, Human Medicine College, Zagazig University, Zagazig, 44631, Egypt
7 Department of Surgery, College of Medicine, Najran University, Najran, 61441, Kingdom of Saudi Arabia
8 Department of Computer Science, Bahauddin Zakariya University, Multan, 66000, Pakistan
9 Radiological Sciences Department, College of Applied Medical Sciences, Najran University, Najran, 61441, Kingdom of Saudi Arabia
* Corresponding Author: Khalaf Alshamrani. Email:
Computers, Materials & Continua 2022, 72(3), 4833-4851. https://doi.org/10.32604/cmc.2022.027111
Received 11 January 2022; Accepted 08 March 2022; Issue published 21 April 2022
Abstract
Breast cancer (BC) is the most common cause of women’s deaths worldwide. The mammography technique is the most important modality for the detection of BC. To detect abnormalities in mammographic images, the Breast Imaging Reporting and Data System (BI-RADs) is used as a baseline. The correct allocation of BI-RADs categories for mammographic images is always an interesting task, even for specialists. In this work, to detect and classify the mammogram images in BI-RADs, a novel hybrid model is presented using a convolutional neural network (CNN) with the integration of a support vector machine (SVM). The dataset used in this research was collected from different hospitals in the Qassim health cluster of Saudi Arabia. The collection of all categories of BI-RADs is one of the major contributions of this paper. Another significant contribution is the development of a hybrid approach through the integration of CNN and SVM. The proposed hybrid approach uses three CNN models to obtain ensemble CNN model results. This ensemble model saves the values to integrate them with SVM. The proposed system achieved a classification accuracy, sensitivity, specificity, precision, and F1-score of 93.6%, 94.8%, 96.9%, 96.6%, and 95.7%, respectively. The proposed model achieved better performance compared to previously available methods.Keywords
Cite This Article
Y. Edrees Almalki1, A. Shaf, T. Ali, M. Aamir, S. Khalid Alduraibi et al., "Breast cancer detection in saudi arabian women using hybrid machine learning on mammographic images," Computers, Materials & Continua, vol. 72, no.3, pp. 4833–4851, 2022. https://doi.org/10.32604/cmc.2022.027111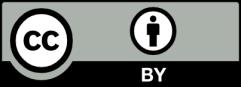