Open Access
ARTICLE
Efficient Feature Selection and Machine Learning Based ADHD Detection Using EEG Signal
1 School of Computer Science and Engineering, The University of Aizu, Aizuwakamatsu, Fukushima, 965-8580, Japan
2 Faculty of Humanities and Social Science, Kumamoto University, Chuo-ku, Kumamoto, Japan
* Corresponding Author: Jungpil Shin. Email:
Computers, Materials & Continua 2022, 72(3), 5179-5195. https://doi.org/10.32604/cmc.2022.028339
Received 08 February 2022; Accepted 16 March 2022; Issue published 21 April 2022
Abstract
Attention deficit hyperactivity disorder (ADHD) is one of the most common psychiatric and neurobehavioral disorders in children, affecting 11% of children worldwide. This study aimed to propose a machine learning (ML)-based algorithm for discriminating ADHD from healthy children using their electroencephalography (EEG) signals. The study included 61 children with ADHD and 60 healthy children aged 7–12 years. Different morphological and time-domain features were extracted from EEG signals. The t-test (p-value < 0.05) and least absolute shrinkage and selection operator (LASSO) were used to select potential features of children with ADHD and enhance the classification accuracy. The selected potential features were used in four ML-based algorithms, including support vector machine (SVM), k-nearest neighbors, multilayer perceptron (MLP), and logisticregression, to classify ADHD and healthy children. The overall prevalence of boys and girls with ADHD was 48.9% and 56.5%, respectively. The average age of children with ADHD was 9.61.8 years. Our results illustrated that the combination of LASSO with SVM classifier achieved the highest accuracy of 94.2%, sensitivity of 93.3%, F1-score of 91.9%, and AUC of 0.964. Our results also illustrated that MLP was the second-best ML-based classifier, which gave 93.4% accuracy, 91.7% sensitivity, 91.1% F1-score, and 0.960 AUC. The findings indicated that the combination of the LASSO-based feature selection method and SVM classifier can be a useful tool for selecting reliable/potential features and classifying ADHD and healthy children. Our proposed ML-based algorithms could be useful for the early diagnosis of children with ADHD.Keywords
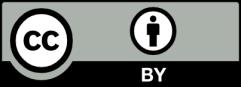