Open Access
ARTICLE
LBP–Bilateral Based Feature Fusion for Breast Cancer Diagnosis
1 Division of Radiology, Department of Medicine, Medical College, Najran University, Najran 61441, Saudi Arabia
2 Department of Computer Science and Information Technology, Ibadat International University, Islamabad, 44000, Pakistan
3 Department of Radiology, College of Medicine, Qassim University, Buraidah 52571, Saudi Arabia
4 Department of Radiology, King Fahad Specialist Hospital, Buraydah 52571, Saudi Arabia
5 Electrical Engineering Department, College of Engineering, Najran University, Najran 61441, Saudi Arabia
6 Radiology Department, Human Medicine College, Zagazig University, Zagazig 44631, Egypt
7 Department of Surgery, College of Medicine, Najran University, Najran 61441, Saudi Arabia
8 Radiological Sciences Department, College of Applied Medical Sciences, Najran University, Najran 61441, Saudi Arabia
* Corresponding Author: Hassan A. Alshamrani. Email:
Computers, Materials & Continua 2022, 73(2), 4103-4121. https://doi.org/10.32604/cmc.2022.029039
Received 23 February 2022; Accepted 06 May 2022; Issue published 16 June 2022
Abstract
Since reporting cases of breast cancer are on the rise all over the world. Especially in regions such as Pakistan, Saudi Arabia, and the United States. Efficient methods for the early detection and diagnosis of breast cancer are needed. The usual diagnosis procedures followed by physicians has been updated with modern diagnostic approaches that include computer-aided support for better accuracy. Machine learning based practices has increased the accuracy and efficiency of medical diagnosis, which has helped save lives of many patients. There is much research in the field of medical imaging diagnostics that can be applied to the variety of data such as magnetic resonance images (MRIs), mammograms, X-rays, ultrasounds, and histopathological images, but magnetic resonance (MR) and mammogram imaging have proved to present the promising results. The proposed paper has presented the results of classification algorithms over Breast Cancer (BC) mammograms from a novel dataset taken from hospitals in the Qassim health cluster of Saudi Arabia. This paper has developed a novel approach called the novel spectral extraction algorithm (NSEA) that uses feature extraction and fusion by using local binary pattern (LBP) and bilateral algorithms, as well as a support vector machine (SVM) as a classifier. The NSEA with the SVM classifier demonstrated a promising accuracy of 94% and an elapsed time of 0.68 milliseconds, which were significantly better results than those of comparative experiments from classifiers named Naïve Bayes, logistic regression, K-Nearest Neighbor (KNN), Gaussian Discriminant Analysis (GDA), AdaBoost and Extreme Learning Machine (ELM). ELM produced the comparative accuracy of 94% however has a lower elapsed time of 1.35 as compared to SVM. Adaboost has produced a fairly well accuracy of 82%, KNN has a low accuracy of 66%. However Logistic Regression, GDA and Naïve Bayes have produced the lowest accuracies of 47%, 43% and 42%.Keywords
Cite This Article
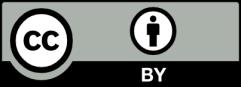