Open Access
ARTICLE
MCBC-SMOTE: A Majority Clustering Model for Classification of Imbalanced Data
1 Department of Information Technology, MSIT, GGSIPU, New Delhi, 110058, India
2 Department of Electrical and Electronic Engineering, MSIT, GGSIPU, New Delhi, 110058, India
3 School of Computer Science and Engineering, Lovely Professional University, 144411, Punjab, India
4 Department of Information Technology, College of Computers and Information Technology, Taif University, 11099, Taif 21944, Saudi Arabia
* Corresponding Author: Aman Singh. Email:
Computers, Materials & Continua 2022, 73(3), 4801-4817. https://doi.org/10.32604/cmc.2022.025960
Received 10 December 2021; Accepted 02 March 2022; Issue published 28 July 2022
Abstract
Datasets with the imbalanced class distribution are difficult to handle with the standard classification algorithms. In supervised learning, dealing with the problem of class imbalance is still considered to be a challenging research problem. Various machine learning techniques are designed to operate on balanced datasets; therefore, the state of the art, different under-sampling, over-sampling and hybrid strategies have been proposed to deal with the problem of imbalanced datasets, but highly skewed datasets still pose the problem of generalization and noise generation during resampling. To over-come these problems, this paper proposes a majority clustering model for classification of imbalanced datasets known as MCBC-SMOTE (Majority Clustering for balanced Classification-SMOTE). The model provides a method to convert the problem of binary classification into a multi-class problem. In the proposed algorithm, the number of clusters for the majority class is calculated using the elbow method and the minority class is over-sampled as an average of clustered majority classes to generate a symmetrical class distribution. The proposed technique is cost-effective, reduces the problem of noise generation and successfully disables the imbalances present in between and within classes. The results of the evaluations on diverse real datasets proved to provide better classification results as compared to state of the art existing methodologies based on several performance metrics.Keywords
Cite This Article
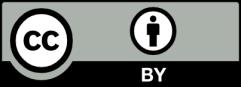