Open Access
ARTICLE
An End-to-End Transformer-Based Automatic Speech Recognition for Qur’an Reciters
1 Department of Information Technology, College of Computer, Qassim University, Buraydah, 51452, Saudi Arabia
2 Department of Computer Science, College of Applied Sciences, Taiz University, Taiz, 6803, Yemen
3 Computer Science Department, Faculty of Computer and Information Sciences, Ain Shams University, Cairo, 11566, Egypt
4 Computer Science Department, Faculty of Sciences, Ibb University, Yemen
5 Department of Computer Sciences & Information, Ibb University, Yemen
* Corresponding Author: Mohammed Hadwan. Email:
Computers, Materials & Continua 2023, 74(2), 3471-3487. https://doi.org/10.32604/cmc.2023.033457
Received 17 June 2022; Accepted 19 August 2022; Issue published 31 October 2022
Abstract
The attention-based encoder-decoder technique, known as the trans-former, is used to enhance the performance of end-to-end automatic speech recognition (ASR). This research focuses on applying ASR end-to-end transformer-based models for the Arabic language, as the researchers’ community pays little attention to it. The Muslims Holy Qur’an book is written using Arabic diacritized text. In this paper, an end-to-end transformer model to building a robust Qur’an vs. recognition is proposed. The acoustic model was built using the transformer-based model as deep learning by the PyTorch framework. A multi-head attention mechanism is utilized to represent the encoder and decoder in the acoustic model. A Mel filter bank is used for feature extraction. To build a language model (LM), the Recurrent Neural Network (RNN) and Long short-term memory (LSTM) were used to train an n-gram word-based LM. As a part of this research, a new dataset of Qur’an verses and their associated transcripts were collected and processed for training and evaluating the proposed model, consisting of 10 h of .wav recitations performed by 60 reciters. The experimental results showed that the proposed end-to-end transformer-based model achieved a significant low character error rate (CER) of 1.98% and a word error rate (WER) of 6.16%. We have achieved state-of-the-art end-to-end transformer-based recognition for Qur’an reciters.Keywords
Cite This Article
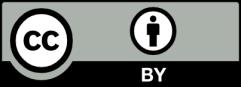