Open Access
ARTICLE
Sailfish Optimizer with Deep Transfer Learning-Enabled Arabic Handwriting Character Recognition
1 Department of Information Systems, College of Computer Science, King Khalid University, Abha, Saudi Arabia
2 Department of Language Preparation, Arabic Language Teaching Institute, Princess Nourah bint Abdulrahman University, P.O. Box 84428, Riyadh, 11671, Saudi Arabia
3 Department of Industrial Engineering, College of Engineering at Alqunfudah, Umm Al-Qura University, Saudi Arabia
4 Department of Computer Science, College of Computing and Information Technology, Shaqra University, Shaqra, Saudi Arabia
5 Prince Saud AlFaisal Institute for Diplomatic Studies, Riyadh, Saudi Arabia
6 Department of Information Technology, College of Computers and Information Technology, Taif University, P.O. Box 11099, Taif, 21944, Saudi Arabia
7 Department of Computer Science, Faculty of Computers and Information Technology, Future University in Egypt, New Cairo, 11835, Egypt
8 Department of Computer and Self Development, Preparatory Year Deanship, Prince Sattam bin Abdulaziz University, AlKharj, Saudi Arabia
* Corresponding Author: Saeed Masoud Alshahrani. Email:
Computers, Materials & Continua 2023, 74(3), 5467-5482. https://doi.org/10.32604/cmc.2023.033534
Received 20 June 2022; Accepted 29 September 2022; Issue published 28 December 2022
Abstract
The recognition of the Arabic characters is a crucial task in computer vision and Natural Language Processing fields. Some major complications in recognizing handwritten texts include distortion and pattern variabilities. So, the feature extraction process is a significant task in NLP models. If the features are automatically selected, it might result in the unavailability of adequate data for accurately forecasting the character classes. But, many features usually create difficulties due to high dimensionality issues. Against this background, the current study develops a Sailfish Optimizer with Deep Transfer Learning-Enabled Arabic Handwriting Character Recognition (SFODTL-AHCR) model. The projected SFODTL-AHCR model primarily focuses on identifying the handwritten Arabic characters in the input image. The proposed SFODTL-AHCR model pre-processes the input image by following the Histogram Equalization approach to attain this objective. The Inception with ResNet-v2 model examines the pre-processed image to produce the feature vectors. The Deep Wavelet Neural Network (DWNN) model is utilized to recognize the handwritten Arabic characters. At last, the SFO algorithm is utilized for fine-tuning the parameters involved in the DWNN model to attain better performance. The performance of the proposed SFODTL-AHCR model was validated using a series of images. Extensive comparative analyses were conducted. The proposed method achieved a maximum accuracy of 99.73%. The outcomes inferred the supremacy of the proposed SFODTL-AHCR model over other approaches.Keywords
Cite This Article
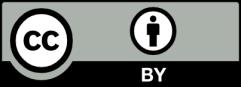