Open Access
ARTICLE
Automated Arabic Text Classification Using Hyperparameter Tuned Hybrid Deep Learning Model
1 Department of Language Preparation, Arabic Language Teaching Institute, Princess Nourah bint Abdulrahman University, P.O. Box 84428, Riyadh, 11671, Saudi Arabia
2 Department of Information Systems, College of Computing and Information System, Umm Al-Qura University, Saudi Arabia
3 Department of Computer Science, College of Computing and Information Technology, Shaqra University, Shaqra, Saudi Arabia
4 Prince Saud AlFaisal Institute for Diplomatic Studies, Saudi Arabia
5 Department of Information Technology, College of Computers and Information Technology, Taif University, Taif P.O. Box 11099, Taif, 21944, Saudi Arabia
6 Department of Electrical Engineering, Faculty of Engineering & Technology, Future University in Egypt, New Cairo, 11845, Egypt
7 Department of Computer and Self Development, Preparatory Year Deanship, Prince Sattam bin Abdulaziz University, AlKharj, Saudi Arabia
* Corresponding Author: Saeed Masoud Alshahrani. Email:
Computers, Materials & Continua 2023, 74(3), 5447-5465. https://doi.org/10.32604/cmc.2023.033564
Received 20 June 2022; Accepted 09 September 2022; Issue published 28 December 2022
Abstract
The text classification process has been extensively investigated in various languages, especially English. Text classification models are vital in several Natural Language Processing (NLP) applications. The Arabic language has a lot of significance. For instance, it is the fourth mostly-used language on the internet and the sixth official language of the United Nations. However, there are few studies on the text classification process in Arabic. A few text classification studies have been published earlier in the Arabic language. In general, researchers face two challenges in the Arabic text classification process: low accuracy and high dimensionality of the features. In this study, an Automated Arabic Text Classification using Hyperparameter Tuned Hybrid Deep Learning (AATC-HTHDL) model is proposed. The major goal of the proposed AATC-HTHDL method is to identify different class labels for the Arabic text. The first step in the proposed model is to pre-process the input data to transform it into a useful format. The Term Frequency-Inverse Document Frequency (TF-IDF) model is applied to extract the feature vectors. Next, the Convolutional Neural Network with Recurrent Neural Network (CRNN) model is utilized to classify the Arabic text. In the final stage, the Crow Search Algorithm (CSA) is applied to fine-tune the CRNN model’s hyperparameters, showing the work’s novelty. The proposed AATC-HTHDL model was experimentally validated under different parameters and the outcomes established the supremacy of the proposed AATC-HTHDL model over other approaches.Keywords
Cite This Article
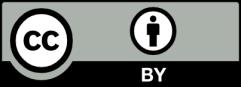