Open Access
ARTICLE
Neural Machine Translation Models with Attention-Based Dropout Layer
1 School of Electrical Engineering and Computer Science (SEECS), National University of Science and Technology, Islamabad, Pakistan
2 Institute for Big Data Analytics and Artificial Intelligence (IBDAAI), Kompleks Al-Khawarizmi, Universiti Teknologi MARA, 40450, Shah Alam, Selangor, Malaysia
* Corresponding Authors: Huma Israr. Email: ; Jasni Mohamad Zain. Email:
Computers, Materials & Continua 2023, 75(2), 2981-3009. https://doi.org/10.32604/cmc.2023.035814
Received 05 September 2022; Accepted 14 December 2022; Issue published 31 March 2023
Abstract
In bilingual translation, attention-based Neural Machine Translation (NMT) models are used to achieve synchrony between input and output sequences and the notion of alignment. NMT model has obtained state-of-the-art performance for several language pairs. However, there has been little work exploring useful architectures for Urdu-to-English machine translation. We conducted extensive Urdu-to-English translation experiments using Long short-term memory (LSTM)/Bidirectional recurrent neural networks (Bi-RNN)/Statistical recurrent unit (SRU)/Gated recurrent unit (GRU)/Convolutional neural network (CNN) and Transformer. Experimental results show that Bi-RNN and LSTM with attention mechanism trained iteratively, with a scalable data set, make precise predictions on unseen data. The trained models yielded competitive results by achieving 62.6% and 61% accuracy and 49.67 and 47.14 BLEU scores, respectively. From a qualitative perspective, the translation of the test sets was examined manually, and it was observed that trained models tend to produce repetitive output more frequently. The attention score produced by Bi-RNN and LSTM produced clear alignment, while GRU showed incorrect translation for words, poor alignment and lack of a clear structure. Therefore, we considered refining the attention-based models by defining an additional attention-based dropout layer. Attention dropout fixes alignment errors and minimizes translation errors at the word level. After empirical demonstration and comparison with their counterparts, we found improvement in the quality of the resulting translation system and a decrease in the perplexity and over-translation score. The ability of the proposed model was evaluated using Arabic-English and Persian-English datasets as well. We empirically concluded that adding an attention-based dropout layer helps improve GRU, SRU, and Transformer translation and is considerably more efficient in translation quality and speed.Keywords
Cite This Article
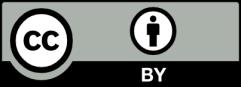