Open Access
ARTICLE
Parameter Tuned Deep Learning Based Traffic Critical Prediction Model on Remote Sensing Imaging
1 Computer Networks Department, Sulaimani Polytechnic University, Sulaimani, Iraq
2 Information Technology Department, Technical College of Informatics-Akre, Duhok Polytechnic University, Iraq
3 Computer Science Department, College of Science, Nawroz University, Duhok, Iraq
4 Energy Eng. Department, Technical College of Engineering, Duhok Polytechnic University, Duhok, Iraq
* Corresponding Author: Subhi R. M. Zeebaree. Email:
Computers, Materials & Continua 2023, 75(2), 3993-4008. https://doi.org/10.32604/cmc.2023.037464
Received 04 November 2022; Accepted 15 January 2023; Issue published 31 March 2023
Abstract
Remote sensing (RS) presents laser scanning measurements, aerial photos, and high-resolution satellite images, which are utilized for extracting a range of traffic-related and road-related features. RS has a weakness, such as traffic fluctuations on small time scales that could distort the accuracy of predicted road and traffic features. This article introduces an Optimal Deep Learning for Traffic Critical Prediction Model on High-Resolution Remote Sensing Images (ODLTCP-HRRSI) to resolve these issues. The presented ODLTCP-HRRSI technique majorly aims to forecast the critical traffic in smart cities. To attain this, the presented ODLTCP-HRRSI model performs two major processes. At the initial stage, the ODLTCP-HRRSI technique employs a convolutional neural network with an auto-encoder (CNN-AE) model for productive and accurate traffic flow. Next, the hyperparameter adjustment of the CNN-AE model is performed via the Bayesian adaptive direct search optimization (BADSO) algorithm. The experimental outcomes demonstrate the enhanced performance of the ODLTCP-HRRSI technique over recent approaches with maximum accuracy of 98.23%.Keywords
Cite This Article
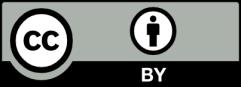