Open Access
ARTICLE
Machine Learning-Enabled Communication Approach for the Internet of Medical Things
1 Faculty of Computing and Informatics, University Malaysia Sabah, Kota Kinabalu, Sabah, 88400, Malaysia
2 Department of Information Technology, College of Computer and Information Sciences, Princess Nourah bint Abdulrahman University, P.O. Box, 84428, Riyadh, 11671, Saudi Arabia
3 Department of Computer Science, Abdul Wali Khan University, Mardan, 23200, Pakistan
4 College of Computer Sciences and Information Technology, King Faisal University, Al-Ahsa, 31982, Saudi Arabia
5 Department of Computer Science and IT, UET Peshawar, Jalozai Campus, Jalozai, 24240, Pakistan
* Corresponding Author: Samia Allaoua Chelloug. Email:
(This article belongs to the Special Issue: IoMT and Smart Healthcare)
Computers, Materials & Continua 2023, 76(2), 1569-1584. https://doi.org/10.32604/cmc.2023.039859
Received 21 February 2023; Accepted 17 May 2023; Issue published 30 August 2023
Abstract
The Internet of Medical Things (IoMT) is mainly concerned with the efficient utilisation of wearable devices in the healthcare domain to manage various processes automatically, whereas machine learning approaches enable these smart systems to make informed decisions. Generally, broadcasting is used for the transmission of frames, whereas congestion, energy efficiency, and excessive load are among the common issues associated with existing approaches. In this paper, a machine learning-enabled shortest path identification scheme is presented to ensure reliable transmission of frames, especially with the minimum possible communication overheads in the IoMT network. For this purpose, the proposed scheme utilises a well-known technique, i.e., Kruskal’s algorithm, to find an optimal path from source to destination wearable devices. Additionally, other evaluation metrics are used to find a reliable and shortest possible communication path between the two interested parties. Apart from that, every device is bound to hold a supplementary path, preferably a second optimised path, for situations where the current communication path is no longer available, either due to device failure or heavy traffic. Furthermore, the machine learning approach helps enable these devices to update their routing tables simultaneously, and an optimal path could be replaced if a better one is available. The proposed mechanism has been tested using a smart environment developed for the healthcare domain using IoMT networks. Simulation results show that the proposed machine learning-oriented approach performs better than existing approaches where the proposed scheme has achieved the minimum possible ratios, i.e., 17% and 23%, in terms of end to end delay and packet losses, respectively. Moreover, the proposed scheme has achieved an approximately 21% improvement in the average throughput compared to the existing schemes.Keywords
Cite This Article
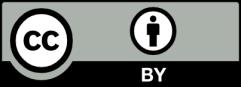