Open Access
ARTICLE
Explainable Artificial Intelligence-Based Model Drift Detection Applicable to Unsupervised Environments
Department of Information Security, Hoseo University, Asan, 31499, Korea
* Corresponding Author: Taejin Lee. Email:
Computers, Materials & Continua 2023, 76(2), 1701-1719. https://doi.org/10.32604/cmc.2023.040235
Received 09 March 2023; Accepted 18 May 2023; Issue published 30 August 2023
Abstract
Cybersecurity increasingly relies on machine learning (ML) models to respond to and detect attacks. However, the rapidly changing data environment makes model life-cycle management after deployment essential. Real-time detection of drift signals from various threats is fundamental for effectively managing deployed models. However, detecting drift in unsupervised environments can be challenging. This study introduces a novel approach leveraging Shapley additive explanations (SHAP), a widely recognized explainability technique in ML, to address drift detection in unsupervised settings. The proposed method incorporates a range of plots and statistical techniques to enhance drift detection reliability and introduces a drift suspicion metric that considers the explanatory aspects absent in the current approaches. To validate the effectiveness of the proposed approach in a real-world scenario, we applied it to an environment designed to detect domain generation algorithms (DGAs). The dataset was obtained from various types of DGAs provided by NetLab. Based on this dataset composition, we sought to validate the proposed SHAP-based approach through drift scenarios that occur when a previously deployed model detects new data types in an environment that detects real-world DGAs. The results revealed that more than 90% of the drift data exceeded the threshold, demonstrating the high reliability of the approach to detect drift in an unsupervised environment. The proposed method distinguishes itself from existing approaches by employing explainable artificial intelligence (XAI)-based detection, which is not limited by model or system environment constraints. In conclusion, this paper proposes a novel approach to detect drift in unsupervised ML settings for cybersecurity. The proposed method employs SHAP-based XAI and a drift suspicion metric to improve drift detection reliability. It is versatile and suitable for various real-time data analysis contexts beyond DGA detection environments. This study significantly contributes to the ML community by addressing the critical issue of managing ML models in real-world cybersecurity settings. Our approach is distinguishable from existing techniques by employing XAI-based detection, which is not limited by model or system environment constraints. As a result, our method can be applied in critical domains that require adaptation to continuous changes, such as cybersecurity. Through extensive validation across diverse settings beyond DGA detection environments, the proposed method will emerge as a versatile drift detection technique suitable for a wide range of real-time data analysis contexts. It is also anticipated to emerge as a new approach to protect essential systems and infrastructures from attacks.Keywords
Cite This Article
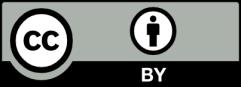