Open Access
ARTICLE
Adaptive Momentum-Backpropagation Algorithm for Flood Prediction and Management in the Internet of Things
1 Department of Computer Science, Muslim Arts College, Manonmaniam Sundaranar University, Thiruvitancode, Tamil Nadu, 629174, India
2 Laboratory Special Communication & Convergence Service Research Center, Kookmin University, Seoul, 02707, Korea
3 Development and Operation Support of Polar Research Equipment, Korea Polar Research Institute (KOPRI), Incheon, 21990, Korea
4 Department of Financial Information Security, Kookmin University, Seoul, 02707, Korea
* Corresponding Author: Soo-Hyun Park. Email:
(This article belongs to the Special Issue: Innovations in Pervasive Computing and Communication Technologies)
Computers, Materials & Continua 2023, 77(1), 1053-1079. https://doi.org/10.32604/cmc.2023.038437
Received 12 December 2022; Accepted 18 August 2023; Issue published 31 October 2023
Abstract
Flooding is a hazardous natural calamity that causes significant damage to lives and infrastructure in the real world. Therefore, timely and accurate decision-making is essential for mitigating flood-related damages. The traditional flood prediction techniques often encounter challenges in accuracy, timeliness, complexity in handling dynamic flood patterns and leading to substandard flood management strategies. To address these challenges, there is a need for advanced machine learning models that can effectively analyze Internet of Things (IoT)-generated flood data and provide timely and accurate flood predictions. This paper proposes a novel approach-the Adaptive Momentum and Backpropagation (AM-BP) algorithm-for flood prediction and management in IoT networks. The AM-BP model combines the advantages of an adaptive momentum technique with the backpropagation algorithm to enhance flood prediction accuracy and efficiency. Real-world flood data is used for validation, demonstrating the superior performance of the AM-BP algorithm compared to traditional methods. In addition, multilayer high-end computing architecture (MLCA) is used to handle weather data such as rainfall, river water level, soil moisture, etc. The AM-BP’s real-time abilities enable proactive flood management, facilitating timely responses and effective disaster mitigation. Furthermore, the AM-BP algorithm can analyze large and complex datasets, integrating environmental and climatic factors for more accurate flood prediction. The evaluation result shows that the AM-BP algorithm outperforms traditional approaches with an accuracy rate of 96%, 96.4% F1-Measure, 97% Precision, and 95.9% Recall. The proposed AM-BP model presents a promising solution for flood prediction and management in IoT networks, contributing to more resilient and efficient flood control strategies, and ensuring the safety and well-being of communities at risk of flooding.Keywords
Cite This Article
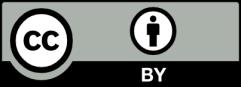