Open Access
ARTICLE
Liver Tumor Prediction with Advanced Attention Mechanisms Integrated into a Depth-Based Variant Search Algorithm
1 Department of Computer Science and Engineering, Saveetha School of Engineering, Chennai, Tamil Nadu, 602117, India
2 Information Technology, Saveetha School of Engineering, Chennai, Tamil Nadu, 602117, India
* Corresponding Author: P. Kalaiselvi. Email:
Computers, Materials & Continua 2023, 77(1), 1209-1226. https://doi.org/10.32604/cmc.2023.040264
Received 11 March 2023; Accepted 01 July 2023; Issue published 31 October 2023
Abstract
In recent days, Deep Learning (DL) techniques have become an emerging transformation in the field of machine learning, artificial intelligence, computer vision, and so on. Subsequently, researchers and industries have been highly endorsed in the medical field, predicting and controlling diverse diseases at specific intervals. Liver tumor prediction is a vital chore in analyzing and treating liver diseases. This paper proposes a novel approach for predicting liver tumors using Convolutional Neural Networks (CNN) and a depth-based variant search algorithm with advanced attention mechanisms (CNN-DS-AM). The proposed work aims to improve accuracy and robustness in diagnosing and treating liver diseases. The anticipated model is assessed on a Computed Tomography (CT) scan dataset containing both benign and malignant liver tumors. The proposed approach achieved high accuracy in predicting liver tumors, outperforming other state-of-the-art methods. Additionally, advanced attention mechanisms were incorporated into the CNN model to enable the identification and highlighting of regions of the CT scans most relevant to predicting liver tumors. The results suggest that incorporating attention mechanisms and a depth-based variant search algorithm into the CNN model is a promising approach for improving the accuracy and robustness of liver tumor prediction. It can assist radiologists in their diagnosis and treatment planning. The proposed system achieved a high accuracy of 95.5% in predicting liver tumors, outperforming other state-of-the-art methods.Keywords
Cite This Article
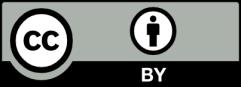