Open Access
ARTICLE
Visualization for Explanation of Deep Learning-Based Fault Diagnosis Model Using Class Activation Map
School of Electrical Engineering, Guizhou University, Guiyang, 550025, China
* Corresponding Author: Qinmu Wu. Email:
(This article belongs to the Special Issue: Advances and Applications in Signal, Image and Video Processing)
Computers, Materials & Continua 2023, 77(2), 1489-1514. https://doi.org/10.32604/cmc.2023.042313
Received 25 May 2023; Accepted 11 August 2023; Issue published 29 November 2023
Abstract
Permanent magnet synchronous motor (PMSM) is widely used in various production processes because of its high efficiency, fast reaction time, and high power density. With the continuous promotion of new energy vehicles, timely detection of PMSM faults can significantly reduce the accident rate of new energy vehicles, further enhance consumers’ trust in their safety, and thus promote their popularity. Existing fault diagnosis methods based on deep learning can only distinguish different PMSM faults and cannot interpret and analyze them. Convolutional neural networks (CNN) show remarkable accuracy in image data analysis. However, due to the “black box” problem in deep learning models, the diagnostic results regarding providing accurate information to the user are uncertain. This paper proposes a motor fault diagnosis method based on improved deep residual network (ResNet) and gradient-weighted class activation mapping (Grad-CAM) to analyze demagnetization and eccentricity faults of permanent magnet synchronous motors, and the uncertainty limitation of fault diagnosis based on the convolutional neural network is overcome by the visual interpretation method. The improved ResNet is formed by using ResNet9 as the backbone network, replacing the last convolution layer with a atrous spatial pyramid pooling (ASPP), and adding a multi-scale feature fusion module and attention channel mechanism (CAM). The proposed model not only retains the effective extraction of image features by ResNet9 but also enhances the sensitivity field of the network through the hollow convolution pyramid and realizes the feature fusion of the web on different scales through the multi-scale feature fusion module (MSFFM), further improving the diagnostic accuracy of the network on different types of fault features. The diagnostic effect of the network is verified on the self-made data set, which mainly includes five states: normal (He), 25% demagnetization (De25), 50% demagnetization (De50), 10% static eccentricity (Se10), and 20% static eccentricity (Se20). The number of pictures in the training set is 6000, and the number in the test set is 1500. The average diagnostic accuracy of the improved ResNet on this dataset is 99.00%, which is 1.04%, 8.89%, 4.58%, and 7.22% higher than that of the multi-column convolutional neural network (MCNN), Bi-directional long short-term memory (Bi-LSTM), deep belief network (DBN), and recurrent neural network (RNN) models, respectively. Finally, gradient activation heat maps were used to globally average pool the final output feature map of the network to obtain feature weights. They were superimposed with the original image to get gradient activation heat maps of different grayscale images. The warmer the tone of the heat map, the greater the impact on the network diagnosis results, and then the demagnetization and eccentricity fault characteristics of the permanent magnet synchronous motor were determined-visual characterization of quantitative analysis.Keywords
Cite This Article
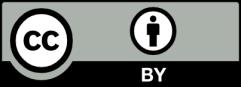