Open Access
ARTICLE
Interactive Transformer for Small Object Detection
Department of Weaponry and Control, Army Academy of Armored Forces, Beijing, 100071, China
* Corresponding Author: Qinzhao Wang. Email:
Computers, Materials & Continua 2023, 77(2), 1699-1717. https://doi.org/10.32604/cmc.2023.044284
Received 26 July 2023; Accepted 15 September 2023; Issue published 29 November 2023
Abstract
The detection of large-scale objects has achieved high accuracy, but due to the low peak signal to noise ratio (PSNR), fewer distinguishing features, and ease of being occluded by the surroundings, the detection of small objects, however, does not enjoy similar success. Endeavor to solve the problem, this paper proposes an attention mechanism based on cross-Key values. Based on the traditional transformer, this paper first improves the feature processing with the convolution module, effectively maintaining the local semantic context in the middle layer, and significantly reducing the number of parameters of the model. Then, to enhance the effectiveness of the attention mask, two Key values are calculated simultaneously along Query and Value by using the method of dual-branch parallel processing, which is used to strengthen the attention acquisition mode and improve the coupling of key information. Finally, focusing on the feature maps of different channels, the multi-head attention mechanism is applied to the channel attention mask to improve the feature utilization effect of the middle layer. By comparing three small object datasets, the plug-and-play interactive transformer (IT-transformer) module designed by us effectively improves the detection results of the baseline.Keywords
Cite This Article
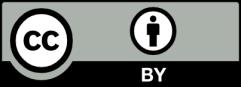