Open Access
ARTICLE
Advancing Brain Tumor Analysis through Dynamic Hierarchical Attention for Improved Segmentation and Survival Prognosis
1 Department of Computer Science and Engineering, Malla Reddy College of Engineering, Secunderabad, Telungana, India
2 Department of Information Technology, Saveetha School of Engineering, Saveetha Institute of Medical and Technical Sciences, SIMATS, Chennai, Tamil Nadu, 602 117, India
* Corresponding Author: S. Kannan. Email:
Computers, Materials & Continua 2023, 77(3), 3835-3851. https://doi.org/10.32604/cmc.2023.042465
Received 31 May 2023; Accepted 24 August 2023; Issue published 26 December 2023
Abstract
Gliomas, the most prevalent primary brain tumors, require accurate segmentation for diagnosis and risk assessment. In this paper, we develop a novel deep learning-based method, the Dynamic Hierarchical Attention for Improved Segmentation and Survival Prognosis (DHA-ISSP) model. The DHA-ISSP model combines a three-band 3D convolutional neural network (CNN) U-Net architecture with dynamic hierarchical attention mechanisms, enabling precise tumor segmentation and survival prediction. The DHA-ISSP model captures fine-grained details and contextual information by leveraging attention mechanisms at multiple levels, enhancing segmentation accuracy. By achieving remarkable results, our approach surpasses 369 competing teams in the 2020 Multimodal Brain Tumor Segmentation Challenge. With a Dice similarity coefficient of 0.89 and a Hausdorff distance of 4.8 mm, the DHA-ISSP model demonstrates its effectiveness in accurately segmenting brain tumors. We also extract radio mic characteristics from the segmented tumor areas using the DHA-ISSP model. By applying cross-validation of decision trees to the selected features, we identify crucial predictors for glioma survival, enabling personalized treatment strategies. Utilizing the DHA-ISSP model and the desired features, we assess patients’ overall survival and categorize survivors into short, mid, in addition to long survivors. The proposed work achieved impressive performance metrics, including the highest accuracy of 0.91, precision of 0.84, recall of 0.92, F1 score of 0.88, specificity of 0.94, sensitivity of 0.92, area under the curve (AUC) value of 0.96, and the lowest mean absolute error value of 0.09 and mean squared error value of 0.18. These results clearly demonstrate the superiority of the proposed system in accurately segmenting brain tumors and predicting survival outcomes, highlighting its significant merit and potential for clinical applications.Keywords
Cite This Article
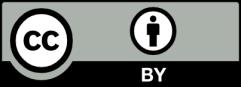