Open Access
ARTICLE
Image Inpainting Technique Incorporating Edge Prior and Attention Mechanism
College of Information Engineering, Xizang Minzu University, Xianyang, 712000, China
* Corresponding Author: Yao Fan. Email:
(This article belongs to the Special Issue: Advances and Applications in Signal, Image and Video Processing)
Computers, Materials & Continua 2024, 78(1), 999-1025. https://doi.org/10.32604/cmc.2023.044612
Received 04 August 2023; Accepted 24 November 2023; Issue published 30 January 2024
Abstract
Recently, deep learning-based image inpainting methods have made great strides in reconstructing damaged regions. However, these methods often struggle to produce satisfactory results when dealing with missing images with large holes, leading to distortions in the structure and blurring of textures. To address these problems, we combine the advantages of transformers and convolutions to propose an image inpainting method that incorporates edge priors and attention mechanisms. The proposed method aims to improve the results of inpainting large holes in images by enhancing the accuracy of structure restoration and the ability to recover texture details. This method divides the inpainting task into two phases: edge prediction and image inpainting. Specifically, in the edge prediction phase, a transformer architecture is designed to combine axial attention with standard self-attention. This design enhances the extraction capability of global structural features and location awareness. It also balances the complexity of self-attention operations, resulting in accurate prediction of the edge structure in the defective region. In the image inpainting phase, a multi-scale fusion attention module is introduced. This module makes full use of multi-level distant features and enhances local pixel continuity, thereby significantly improving the quality of image inpainting. To evaluate the performance of our method, comparative experiments are conducted on several datasets, including CelebA, Places2, and Facade. Quantitative experiments show that our method outperforms the other mainstream methods. Specifically, it improves Peak Signal-to-Noise Ratio (PSNR) and Structure Similarity Index Measure (SSIM) by 1.141~3.234 db and 0.083~0.235, respectively. Moreover, it reduces Learning Perceptual Image Patch Similarity (LPIPS) and Mean Absolute Error (MAE) by 0.0347~0.1753 and 0.0104~0.0402, respectively. Qualitative experiments reveal that our method excels at reconstructing images with complete structural information and clear texture details. Furthermore, our model exhibits impressive performance in terms of the number of parameters, memory cost, and testing time.Keywords
Cite This Article
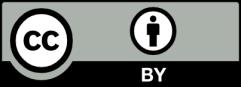