Open Access
ARTICLE
Dynamic Routing of Multiple QoS-Required Flows in Cloud-Edge Autonomous Multi-Domain Data Center Networks
1 School of Computer Science (National Pilot Software Engineering School), Beijing University of Posts and Telecommunications, Beijing, 100876, China
2 College of Computer Science and Technology, Tianjin University, Tianjin, 300072, China
3 Institute of Artificial Intelligence, Nankai University, Tianjin, 300071, China
4 College of Information Science and Engineering, East China University of Science and Technology, Shanghai, 200237, China
* Corresponding Author: Shiyan Zhang. Email:
(This article belongs to the Special Issue: Transfroming from Data to Knowledge and Applications in Intelligent Systems)
Computers, Materials & Continua 2024, 78(2), 2287-2308. https://doi.org/10.32604/cmc.2023.046550
Received 06 October 2023; Accepted 23 November 2023; Issue published 27 February 2024
Abstract
The 6th generation mobile networks (6G) network is a kind of multi-network interconnection and multi-scenario coexistence network, where multiple network domains break the original fixed boundaries to form connections and convergence. In this paper, with the optimization objective of maximizing network utility while ensuring flows performance-centric weighted fairness, this paper designs a reinforcement learning-based cloud-edge autonomous multi-domain data center network architecture that achieves single-domain autonomy and multi-domain collaboration. Due to the conflict between the utility of different flows, the bandwidth fairness allocation problem for various types of flows is formulated by considering different defined reward functions. Regarding the tradeoff between fairness and utility, this paper deals with the corresponding reward functions for the cases where the flows undergo abrupt changes and smooth changes in the flows. In addition, to accommodate the Quality of Service (QoS) requirements for multiple types of flows, this paper proposes a multi-domain autonomous routing algorithm called LSTM+MADDPG. Introducing a Long Short-Term Memory (LSTM) layer in the actor and critic networks, more information about temporal continuity is added, further enhancing the adaptive ability changes in the dynamic network environment. The LSTM+MADDPG algorithm is compared with the latest reinforcement learning algorithm by conducting experiments on real network topology and traffic traces, and the experimental results show that LSTM+MADDPG improves the delay convergence speed by 14.6% and delays the start moment of packet loss by 18.2% compared with other algorithms.Keywords
Cite This Article
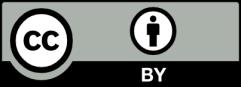