Open Access
ARTICLE
Improving Thyroid Disorder Diagnosis via Ensemble Stacking and Bidirectional Feature Selection
1 Department of Computer and Information System, Cleveland State University, Ohio, 44115, USA
2 Department of Electrical, Electronics and Computer Systems, College of Engineering and Technology, University of Sargodha, Sargodha, 40100, Pakistan
3 Department of Mechanical Engineering, College of Engineering, King Faisal University, Al-Ahsa, 31982, Saudi Arabia
4 Department of Biomedical Engineering, Riphah International University, Islamabad, 44000, Pakistan
5 Department of Electrical Engineering, Riphah International University, Islamabad, 44000, Pakistan
6 Department of Information Technology, College of Computer and Information Sciences, Princess Nourah bint Abdulrahman University, P.O. Box 84428, Riyadh, 11671, Saudi Arabia
7 Department of Artificial Intelligence, College of Software and Convergence Technology, Sejong University, Seoul, 05006, Korea
* Corresponding Author: Maali Alabdulhafith. Email:
(This article belongs to the Special Issue: Privacy-Aware AI-based Models for Cancer Diagnosis)
Computers, Materials & Continua 2024, 78(3), 4225-4241. https://doi.org/10.32604/cmc.2024.047621
Received 11 November 2023; Accepted 01 February 2024; Issue published 26 March 2024
Abstract
Thyroid disorders represent a significant global health challenge with hypothyroidism and hyperthyroidism as two common conditions arising from dysfunction in the thyroid gland. Accurate and timely diagnosis of these disorders is crucial for effective treatment and patient care. This research introduces a comprehensive approach to improve the accuracy of thyroid disorder diagnosis through the integration of ensemble stacking and advanced feature selection techniques. Sequential forward feature selection, sequential backward feature elimination, and bidirectional feature elimination are investigated in this study. In ensemble learning, random forest, adaptive boosting, and bagging classifiers are employed. The effectiveness of these techniques is evaluated using two different datasets obtained from the University of California Irvine-Machine Learning Repository, both of which undergo preprocessing steps, including outlier removal, addressing missing data, data cleansing, and feature reduction. Extensive experimentation demonstrates the remarkable success of proposed ensemble stacking and bidirectional feature elimination achieving 100% and 99.86% accuracy in identifying hyperthyroidism and hypothyroidism, respectively. Beyond enhancing detection accuracy, the ensemble stacking model also demonstrated a streamlined computational complexity which is pivotal for practical medical applications. It significantly outperformed existing studies with similar objectives underscoring the viability and effectiveness of the proposed scheme. This research offers an innovative perspective and sets the platform for improved thyroid disorder diagnosis with broader implications for healthcare and patient well-being.Keywords
Cite This Article
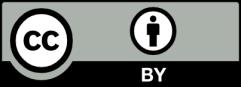