Open Access
ARTICLE
Hybrid Sooty Tern Optimization and Differential Evolution for Feature Selection
1 College of Information Engineering, Sanming University, Sanming, 365004, China
2 College of Mechanical and Electrical Engineering, Northeast Forestry University, Harbin, 150040, China
3 School of Engineering, Manukau Institute of Technology, Auckland, 2241, New Zealand
* Corresponding Author: Heming Jia. Email:
Computer Systems Science and Engineering 2021, 39(3), 321-335. https://doi.org/10.32604/csse.2021.017536
Received 02 February 2021; Accepted 20 March 2021; Issue published 12 August 2021
Abstract
In this paper, a hybrid model based on sooty tern optimization algorithm (STOA) is proposed to optimize the parameters of the support vector machine (SVM) and identify the best feature sets simultaneously. Feature selection is an essential process of data preprocessing, and it aims to find the most relevant subset of features. In recent years, it has been applied in many practical domains of intelligent systems. The application of SVM in many fields has proved its effectiveness in classification tasks of various types. Its performance is mainly determined by the kernel type and its parameters. One of the most challenging process in machine learning is feature selection, intending to select effective and representative features. The main disadvantages of feature selection processes included in classical optimization algorithm are local optimal stagnation and slow convergence. Therefore, the hybrid model proposed in this paper merges the STOA and differential evolution (DE) to improve the search efficiency and convergence rate. A series of experiments are conducted on 12 datasets from the UCI repository to comprehensively and objectively evaluate the performance of the proposed method. The superiority of the proposed method is illustrated from different aspects, such as the classification accuracy, convergence performance, reduced feature dimensionality, standard deviation (STD), and computation time.Keywords
Cite This Article
Citations
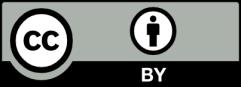