Open Access
ARTICLE
Facial Expression Recognition Using Enhanced Convolution Neural Network with Attention Mechanism
1 Department of EIE, Kongu Engineering College, Perundurai, 638060, Tamilnadu, India
2 Department of EEE, M.Kumarasamy College of Engineering, Karur, 639113, Tamilnadu, India
3 Department of EEE, Kongu Engineering College, Perundurai, 638060, Tamilnadu, India
4 Department of EEE, CSI Engineering College, Ketti, 643215, Tamilnadu, India
* Corresponding Author: K. Prabhu. Email:
Computer Systems Science and Engineering 2022, 41(1), 415-426. https://doi.org/10.32604/csse.2022.019749
Received 24 April 2021; Accepted 14 June 2021; Issue published 08 October 2021
Abstract
Facial Expression Recognition (FER) has been an interesting area of research in places where there is human-computer interaction. Human psychology, emotions and behaviors can be analyzed in FER. Classifiers used in FER have been perfect on normal faces but have been found to be constrained in occluded faces. Recently, Deep Learning Techniques (DLT) have gained popularity in applications of real-world problems including recognition of human emotions. The human face reflects emotional states and human intentions. An expression is the most natural and powerful way of communicating non-verbally. Systems which form communications between the two are termed Human Machine Interaction (HMI) systems. FER can improve HMI systems as human expressions convey useful information to an observer. This paper proposes a FER scheme called EECNN (Enhanced Convolution Neural Network with Attention mechanism) to recognize seven types of human emotions with satisfying results in its experiments. Proposed EECNN achieved 89.8% accuracy in classifying the images.Keywords
Cite This Article
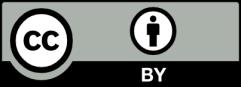