Open Access
ARTICLE
Detection of Parkinson’s Disease with Multiple Feature Extraction Models and Darknet CNN Classification
1 Department of Information Technology, Kumaraguru College of Technology, Coimbatore, 641049, India
2 Department of Computer Science and Engineering, Kumaraguru College of Technology, Coimbatore, 641049, India
* Corresponding Author: G. Prema Arokia Mary. Email:
Computer Systems Science and Engineering 2022, 43(1), 333-345. https://doi.org/10.32604/csse.2022.021164
Received 25 June 2021; Accepted 17 September 2021; Issue published 23 March 2022
Abstract
Parkinson’s disease (PD) is a neurodegenerative disease in the central nervous system. Recently, more researches have been conducted in the determination of PD prediction which is really a challenging task. Due to the disorders in the central nervous system, the syndromes like off sleep, speech disorders, olfactory and autonomic dysfunction, sensory disorder symptoms will occur. The earliest diagnosing of PD is very challenging among the doctors community. There are techniques that are available in order to predict PD using symptoms and disorder measurement. It helps to save a million lives of future by early prediction. In this article, the early diagnosing of PD using machine learning techniques with feature selection is carried out. In the first stage, the data preprocessing is used for the preparation of Parkinson’s disease data. In the second stage, MFEA is used for extracting features. In the third stage, the feature selection is performed using multiple feature input with a principal component analysis (PCA) algorithm. Finally, a Darknet Convolutional Neural Network (DNetCNN) is used to classify the PD patients. The main advantage of using PCA- DNetCNN is that, it provides the best classification in the image dataset using YOLO. In addition to that, the results of various existing methods are compared and the proposed DNetCNN proves better accuracy, performance in detecting the PD at the initial stages. DNetCNN achieves 97.5 % of accuracy in detecting PD as early. Besides, the other performance metrics are compared in the result evaluation and it is proved that the proposed model outperforms all the other existing models.Keywords
Cite This Article
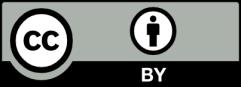