Open Access
ARTICLE
Transfer Learning on Deep Neural Networks to Detect Pornography
Department of Information Technology, College of Computer, Qassim University, Buraydah, Saudi Arabia
* Corresponding Author: Saleh Albahli. Email:
Computer Systems Science and Engineering 2022, 43(2), 701-717. https://doi.org/10.32604/csse.2022.022723
Received 17 August 2021; Accepted 25 November 2021; Issue published 20 April 2022
Abstract
While the internet has a lot of positive impact on society, there are negative components. Accessible to everyone through online platforms, pornography is, inducing psychological and health related issues among people of all ages. While a difficult task, detecting pornography can be the important step in determining the porn and adult content in a video. In this paper, an architecture is proposed which yielded high scores for both training and testing. This dataset was produced from 190 videos, yielding more than 19 h of videos. The main sources for the content were from YouTube, movies, torrent, and websites that hosts both pornographic and non-pornographic contents. The videos were from different ethnicities and skin color which ensures the models can detect any kind of video. A VGG16, Inception V3 and Resnet 50 models were initially trained to detect these pornographic images but failed to achieve a high testing accuracy with accuracies of 0.49, 0.49 and 0.78 respectively. Finally, utilizing transfer learning, a convolutional neural network was designed and yielded an accuracy of 0.98.Keywords
Cite This Article
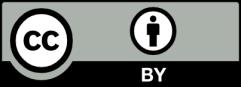