Open Access
ARTICLE
Deep-BERT: Transfer Learning for Classifying Multilingual Offensive Texts on Social Media
1 Department of Computer Science and Engineering, Bangladesh University of Business and Technology, Dhaka, Bangladesh
2 School of Computer Science and Engineering, University of Aizu, Aizuwakamatsu, Japan
3 Department of Computer Science, American International University-Bangladesh, Dhaka, Bangladesh
4 Department of Computer Science and Engineering, University of Asia Pacific, Dhaka, Bangladesh
* Corresponding Author: Jungpil Shin. Email:
Computer Systems Science and Engineering 2023, 44(2), 1775-1791. https://doi.org/10.32604/csse.2023.027841
Received 26 January 2022; Accepted 11 March 2022; Issue published 15 June 2022
Abstract
Offensive messages on social media, have recently been frequently used to harass and criticize people. In recent studies, many promising algorithms have been developed to identify offensive texts. Most algorithms analyze text in a unidirectional manner, where a bidirectional method can maximize performance results and capture semantic and contextual information in sentences. In addition, there are many separate models for identifying offensive texts based on monolingual and multilingual, but there are a few models that can detect both monolingual and multilingual-based offensive texts. In this study, a detection system has been developed for both monolingual and multilingual offensive texts by combining deep convolutional neural network and bidirectional encoder representations from transformers (Deep-BERT) to identify offensive posts on social media that are used to harass others. This paper explores a variety of ways to deal with multilingualism, including collaborative multilingual and translation-based approaches. Then, the Deep-BERT is tested on the Bengali and English datasets, including the different bidirectional encoder representations from transformers (BERT) pre-trained word-embedding techniques, and found that the proposed Deep-BERT’s efficacy outperformed all existing offensive text classification algorithms reaching an accuracy of 91.83%. The proposed model is a state-of-the-art model that can classify both monolingual-based and multilingual-based offensive texts.Keywords
Cite This Article
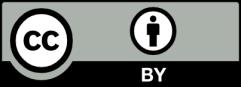