Open Access
ARTICLE
Spotted Hyena-Bat Optimized Extreme Learning Machine for Solar Power Extraction
1 Faculty of Electrical and Electronics Engineering, Mahendra Institute of Technology, Namakkal, Tamilnadu, India
2 Faculty of Electrical and Electronics Engineering, Sona College of Technology, Salem, Tamilnadu, India
* Corresponding Author: K. Madumathi. Email:
Computer Systems Science and Engineering 2023, 45(2), 1821-1836. https://doi.org/10.32604/csse.2023.029561
Received 07 March 2022; Accepted 06 June 2022; Issue published 03 November 2022
Abstract
Artificial intelligence, machine learning and deep learning algorithms have been widely used for Maximum Power Point Tracking (MPPT) in solar systems. In the traditional MPPT strategies, following of worldwide Global Maximum Power Point (GMPP) under incomplete concealing conditions stay overwhelming assignment and tracks different nearby greatest power focuses under halfway concealing conditions. The advent of artificial intelligence in MPPT has guaranteed of accurate following of GMPP while expanding the significant performance and efficiency of MPPT under Partial Shading Conditions (PSC). Still the selection of an efficient learning based MPPT is complex because each model has its advantages and drawbacks. Recently, Meta-heuristic algorithm based Learning techniques have provided better tracking efficiency but still exhibit dull performances under PSC. This work represents an excellent optimization based on Spotted Hyena Enabled Reliable BAT (SHERB) learning models, SHERB-MPPT integrated with powerful extreme learning machines to identify the GMPP with fast convergence, low steady-state oscillations, and good tracking efficiency. Extensive testing using MATLAB-SIMULINK, with 50000 data combinations gathered under partial shade and normal settings. As a result of simulations, the proposed approach offers 99.7% tracking efficiency with a slower convergence speed. To demonstrate the predominance of the proposed system, we have compared the performance of the system with other hybrid MPPT learning models. Results proved that the proposed cross breed MPPT model had beaten different techniques in recognizing GMPP viably under fractional concealing conditions.Keywords
Cite This Article
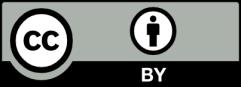