Open Access
ARTICLE
Adaptive Weighted Flow Net Algorithm for Human Activity Recognition Using Depth Learned Features
Department of Information Science and Technology, Anna University, Chennai, 600025, Tamilnadu, India
* Corresponding Author: G. Augusta Kani. Email:
Computer Systems Science and Engineering 2023, 46(2), 1447-1469. https://doi.org/10.32604/csse.2023.035969
Received 12 September 2022; Accepted 25 November 2022; Issue published 09 February 2023
Abstract
Human Activity Recognition (HAR) from video data collections is the core application in vision tasks and has a variety of utilizations including object detection applications, video-based behavior monitoring, video classification, and indexing, patient monitoring, robotics, and behavior analysis. Although many techniques are available for HAR in video analysis tasks, most of them are not focusing on behavioral analysis. Hence, a new HAR system analysis the behavioral activity of a person based on the deep learning approach proposed in this work. The most essential aim of this work is to recognize the complex activities that are useful in many tasks that are based on object detection, modelling of individual frame characteristics, and communication among them. Moreover, this work focuses on finding out the human actions from various video resolutions, invariant human poses, and nearness of multi objects. First, we identify the key and essential frames of each activity using histogram differences. Secondly, Discrete Wavelet Transform (DWT) is used in this system to extract coefficients from the sequence of key-frames where the activity is localized in space. Finally, an Adaptive Weighted Flow Net (AWFN) algorithm is proposed in this work for effective video activity recognition. Moreover, the proposed algorithm has been evaluated by comparing it with the existing Visual Geometry Group (VGG-16) convolution neural networks for making performance comparisons. This work focuses on competent deep learning-based feature extraction to discriminate the activities for performing the classification accuracy. The proposed model has been evaluated with VGG-16 using a combination of regular UCF-101 activity datasets and also in very challenging Low-quality videos such as HMDB51. From these investigations, it is proved that the proposed AWFN approach gives higher detection accuracy of 96%. It is approximately 0.3% to 7.88% of higher accuracy than state-of-art methods.Keywords
Cite This Article
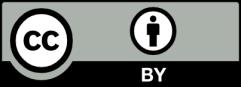