Open Access
ARTICLE
Optimal Quad Channel Long Short-Term Memory Based Fake News Classification on English Corpus
1 Department of Computer and Self Development, Preparatory Year Deanship, Prince Sattam bin Abdulaziz University, AlKharj, Saudi Arabia
2 Department of Applied Linguistics, College of Languages, Princess Nourah bint Abdulrahman University, P.O. Box 84428, Riyadh, 11671, Saudi Arabia
3 Department of Computer Sciences, College of Computing and Information System, Umm Al-Qura University, Saudi Arabia
4 Department of Information Systems, Faculty of Computing and Information Technology, King Abdulaziz University, Jeddah, Saudi Arabia
5 Department of Digital Media, Faculty of Computers and Information Technology, Future University in Egypt, New Cairo, 11845, Egypt
6 Department of Information System, College of Computer Engineering and Sciences, Prince Sattam bin Abdulaziz University, AlKharj, Saudi Arabia
* Corresponding Author: Manar Ahmed Hamza. Email:
Computer Systems Science and Engineering 2023, 46(3), 3303-3319. https://doi.org/10.32604/csse.2023.034823
Received 28 July 2022; Accepted 07 November 2022; Issue published 03 April 2023
Abstract
The term ‘corpus’ refers to a huge volume of structured datasets containing machine-readable texts. Such texts are generated in a natural communicative setting. The explosion of social media permitted individuals to spread data with minimal examination and filters freely. Due to this, the old problem of fake news has resurfaced. It has become an important concern due to its negative impact on the community. To manage the spread of fake news, automatic recognition approaches have been investigated earlier using Artificial Intelligence (AI) and Machine Learning (ML) techniques. To perform the medicinal text classification tasks, the ML approaches were applied, and they performed quite effectively. Still, a huge effort is required from the human side to generate the labelled training data. The recent progress of the Deep Learning (DL) methods seems to be a promising solution to tackle difficult types of Natural Language Processing (NLP) tasks, especially fake news detection. To unlock social media data, an automatic text classifier is highly helpful in the domain of NLP. The current research article focuses on the design of the Optimal Quad Channel Hybrid Long Short-Term Memory-based Fake News Classification (QCLSTM-FNC) approach. The presented QCLSTM-FNC approach aims to identify and differentiate fake news from actual news. To attain this, the proposed QCLSTM-FNC approach follows two methods such as the pre-processing data method and the Glove-based word embedding process. Besides, the QCLSTM model is utilized for classification. To boost the classification results of the QCLSTM model, a Quasi-Oppositional Sandpiper Optimization (QOSPO) algorithm is utilized to fine-tune the hyperparameters. The proposed QCLSTM-FNC approach was experimentally validated against a benchmark dataset. The QCLSTM-FNC approach successfully outperformed all other existing DL models under different measures.Keywords
Cite This Article
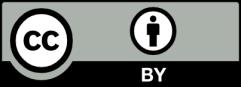