Open Access
ARTICLE
Spotted Hyena Optimizer Driven Deep Learning-Based Drug-Drug Interaction Prediction in Big Data Environment
1 Engineering College, Al-Kitab University, Kirkuk, Iraq
2 Information Technology Department, Technical College of Informatics-Akre, Duhok Polytechnic University, Duhok, Iraq
3 Computer Science Department, College of Science, Nawroz University, Duhok, Iraq
4 Energy Engineering Department, Technical College of Engineering, Duhok Polytechnic University, Duhok, Iraq
* Corresponding Author: Subhi R. M. Zeebaree. Email:
Computer Systems Science and Engineering 2023, 46(3), 3831-3845. https://doi.org/10.32604/csse.2023.037580
Received 09 November 2022; Accepted 09 February 2023; Issue published 03 April 2023
Abstract
Nowadays, smart healthcare and biomedical research have marked a substantial growth rate in terms of their presence in the literature, computational approaches, and discoveries, owing to which a massive quantity of experimental datasets was published and generated (Big Data) for describing and validating such novelties. Drug-drug interaction (DDI) significantly contributed to drug administration and development. It continues as the main obstacle in offering inexpensive and safe healthcare. It normally happens for patients with extensive medication, leading them to take many drugs simultaneously. DDI may cause side effects, either mild or severe health problems. This reduced victims’ quality of life and increased hospital healthcare expenses by increasing their recovery time. Several efforts were made to formulate new methods for DDI prediction to overcome this issue. In this aspect, this study designs a new Spotted Hyena Optimizer Driven Deep Learning based Drug-Drug Interaction Prediction (SHODL-DDIP) model in a big data environment. In the presented SHODL-DDIP technique, the relativity and characteristics of the drugs can be identified from different sources for prediction. The input data is preprocessed at the primary level to improve its quality. Next, the salp swarm optimization algorithm (SSO) is used to select features. In this study, the deep belief network (DBN) model is exploited to predict the DDI accurately. The SHO algorithm is involved in improvising the DBN model’s predictive outcomes, showing the novelty of the work. The experimental result analysis of the SHODL-DDIP technique is tested using drug databases, and the results signified the improvements of the SHODL-DDIP technique over other recent models in terms of different performance measures.Keywords
Cite This Article
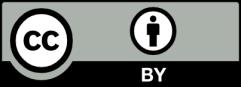