Open Access
REVIEW
A Survey on Acute Leukemia Expression Data Classification Using Ensembles
1 Department of Information Systems, Faculty of Computer Science, Misr International University, Cairo, 11828, Egypt
2 Department of Information Technology, Faculty of Computers and Informatics, Zagazig University, Zagazig, 44516, Egypt
3 Department of Information Systems, Faculty of Computers and Informatics, Zagazig University, Zagazig, 44516, Egypt
* Corresponding Author: Mona Gamal. Email:
Computer Systems Science and Engineering 2023, 47(2), 1349-1364. https://doi.org/10.32604/csse.2023.033596
Received 21 June 2022; Accepted 23 November 2022; Issue published 28 July 2023
Abstract
Acute leukemia is an aggressive disease that has high mortality rates worldwide. The error rate can be as high as 40% when classifying acute leukemia into its subtypes. So, there is an urgent need to support hematologists during the classification process. More than two decades ago, researchers used microarray gene expression data to classify cancer and adopted acute leukemia as a test case. The high classification accuracy they achieved confirmed that it is possible to classify cancer subtypes using microarray gene expression data. Ensemble machine learning is an effective method that combines individual classifiers to classify new samples. Ensemble classifiers are recognized as powerful algorithms with numerous advantages over traditional classifiers. Over the past few decades, researchers have focused a great deal of attention on ensemble classifiers in a wide variety of fields, including but not limited to disease diagnosis, finance, bioinformatics, healthcare, manufacturing, and geography. This paper reviews the recent ensemble classifier approaches utilized for acute leukemia gene expression data classification. Moreover, a framework for classifying acute leukemia gene expression data is proposed. The pairwise correlation gene selection method and the Rotation Forest of Bayesian Networks are both used in this framework. Experimental outcomes show that the classification accuracy achieved by the acute leukemia ensemble classifiers constructed according to the suggested framework is good compared to the classification accuracy achieved in other studies.Keywords
Cite This Article
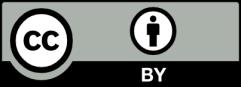