Open Access
ARTICLE
Fast and Accurate Detection of Masked Faces Using CNNs and LBPs
1 Department of Computer Sciences, College of Computer and Information Sciences, Princess Nourah bint Abdulrahman University, P.O. Box 84428, Riyadh, 11671, Saudi Arabia
2 Computer Science Department, Sinai University, Arish, North Sinai, Egypt
3 Department of Information Technology, Faculty of Computers and Informatics, Zagazig University, Zagazig, Egypt
* Corresponding Author: Doaa Sami Khafaga. Email:
Computer Systems Science and Engineering 2023, 47(3), 2939-2952. https://doi.org/10.32604/csse.2023.041011
Received 07 April 2023; Accepted 25 July 2023; Issue published 09 November 2023
Abstract
Face mask detection has several applications, including real-time surveillance, biometrics, etc. Identifying face masks is also helpful for crowd control and ensuring people wear them publicly. With monitoring personnel, it is impossible to ensure that people wear face masks; automated systems are a much superior option for face mask detection and monitoring. This paper introduces a simple and efficient approach for masked face detection. The architecture of the proposed approach is very straightforward; it combines deep learning and local binary patterns to extract features and classify them as masked or unmasked. The proposed system requires hardware with minimal power consumption compared to state-of-the-art deep learning algorithms. Our proposed system maintains two steps. At first, this work extracted the local features of an image by using a local binary pattern descriptor, and then we used deep learning to extract global features. The proposed approach has achieved excellent accuracy and high performance. The performance of the proposed method was tested on three benchmark datasets: the real-world masked faces dataset (RMFD), the simulated masked faces dataset (SMFD), and labeled faces in the wild (LFW). Performance metrics for the proposed technique were measured in terms of accuracy, precision, recall, and F1-score. Results indicated the efficiency of the proposed technique, providing accuracies of 99.86%, 99.98%, and 100% for RMFD, SMFD, and LFW, respectively. Moreover, the proposed method outperformed state-of-the-art deep learning methods in the recent bibliography for the same problem under study and on the same evaluation datasets.Keywords
Cite This Article
S. M. Alhammad, D. S. Khafaga, A. Y. Hamed, O. El-Koumy, E. R. Mohamed et al., "Fast and accurate detection of masked faces using cnns and lbps," Computer Systems Science and Engineering, vol. 47, no.3, pp. 2939–2952, 2023. https://doi.org/10.32604/csse.2023.041011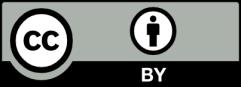