Open Access
ARTICLE
Inferential Statistics and Machine Learning Models for Short-Term Wind Power Forecasting
School of Computer Science, Jiangsu University of Science and Technology, Zhenjiang, 212003, China
* Corresponding Author: Xing Deng. Email:
Energy Engineering 2022, 119(1), 237-252. https://doi.org/10.32604/EE.2022.017916
Received 16 June 2021; Accepted 19 August 2021; Issue published 22 November 2021
Abstract
The inherent randomness, intermittence and volatility of wind power generation compromise the quality of the wind power system, resulting in uncertainty in the system's optimal scheduling. As a result, it's critical to improve power quality and assure real-time power grid scheduling and grid-connected wind farm operation. Inferred statistics are utilized in this research to infer general features based on the selected information, confirming that there are differences between two forecasting categories: Forecast Category 1 (0–11 h ahead) and Forecast Category 2 (12–23 h ahead). In z-tests, the null hypothesis provides the corresponding quantitative findings. To verify the final performance of the prediction findings, five benchmark methodologies are used: Persistence model, LMNN (Multilayer Perceptron with LM learning methods), NARX (Nonlinear autoregressive exogenous neural network model), LMRNN (RNNs with LM training methods) and LSTM (Long short-term memory neural network). Experiments using a real dataset show that the LSTM network has the highest forecasting accuracy when compared to other benchmark approaches including persistence model, LMNN, NARX network, and LMRNN, and the 23-steps forecasting accuracy has improved by 19.61%.Keywords
Cite This Article
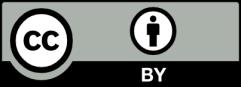