Open Access
ARTICLE
Short-Term Wind Power Prediction Based on ICEEMDAN-SE-LSTM Neural Network Model with Classifying Seasonal
1 State Grid Shandong Electric Power Research Institute, Jinan, 250002, China
2 Key Laboratory of Control of Power Transmission and Conversion, Shanghai Jiao Tong University, Shanghai, 200240, China
* Corresponding Author: Qian Ai. Email:
(This article belongs to the Special Issue: Fault Diagnosis and State Evaluation of New Power Grid)
Energy Engineering 2023, 120(12), 2761-2782. https://doi.org/10.32604/ee.2023.042635
Received 06 June 2023; Accepted 03 August 2023; Issue published 29 November 2023
Abstract
Wind power prediction is very important for the economic dispatching of power systems containing wind power. In this work, a novel short-term wind power prediction method based on improved complete ensemble empirical mode decomposition with adaptive noise (ICEEMDAN) and (long short-term memory) LSTM neural network is proposed and studied. First, the original data is prepossessed including removing outliers and filling in the gaps. Then, the random forest algorithm is used to sort the importance of each meteorological factor and determine the input climate characteristics of the forecast model. In addition, this study conducts seasonal classification of the annual data where ICEEMDAN is adopted to divide the original wind power sequence into numerous modal components according to different seasons. On this basis, sample entropy is used to calculate the complexity of each component and reconstruct them into trend components, oscillation components, and random components. Then, these three components are input into the LSTM neural network, respectively. Combined with the predicted values of the three components, the overall power prediction results are obtained. The simulation shows that ICEEMDAN-SE-LSTM achieves higher prediction accuracy ranging from 1.57% to 9.46% than other traditional models, which indicates the reliability and effectiveness of the proposed method for power prediction.Keywords
Cite This Article
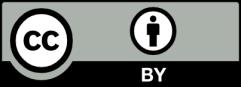