Open Access
ARTICLE
Prediction Model of Drilling Costs for Ultra-Deep Wells Based on GA-BP Neural Network
1
Petroleum Engineering College, Yangtze University, Wuhan, 430199, China
2
PetroChina Tarim Oilfield Company, Korla, 841000, China
3
Hubei Drilling and Recovery Engineering for Oil and Gas Key Laboratory, Wuhan, 430199, China
* Corresponding Author: Dehua Liu. Email:
Energy Engineering 2023, 120(7), 1701-1715. https://doi.org/10.32604/ee.2023.027703
Received 10 November 2022; Accepted 14 February 2023; Issue published 04 May 2023
Abstract
Drilling costs of ultra-deep well is the significant part of development investment, and accurate prediction of drilling costs plays an important role in reasonable budgeting and overall control of development cost. In order to improve the prediction accuracy of ultra-deep well drilling costs, the item and the dominant factors of drilling costs in Tarim oilfield are analyzed. Then, those factors of drilling costs are separated into categorical variables and numerous variables. Finally, a BP neural network model with drilling costs as the output is established, and hyper-parameters (initial weights and bias) of the BP neural network is optimized by genetic algorithm (GA). Through training and validation of the model, a reliable prediction model of ultra-deep well drilling costs is achieved. The average relative error between prediction and actual values is 3.26%. Compared with other models, the root mean square error is reduced by 25.38%. The prediction results of the proposed model are reliable, and the model is efficient, which can provide supporting for the drilling costs control and budget planning of ultra-deep wells.Keywords
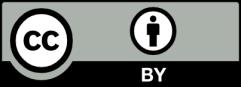