Open Access
ARTICLE
Design and Development of an Intelligent Energy Management System for a Smart Grid to Enhance the Power Quality
1 Department of Electrical and Electronics Engineering, Kalasalingam Academy of Research and Education, Tamil Nadu, India
2 Department of Computer Science Engineering, Kalasalingam Academy of Research and Education, Tamil Nadu, India
3 Department of Electrical and Electronics Engineering, Kongunadu College of Engineering and Technology, Tamil Nadu, India
4 Department of Electrical and Electronics Engineering, Kongu Engineering College, Tamil Nadu, India
5 Department of Electrical and Electronics Engineering, Narasu’s Sarathy Institute of Technology, Tamil Nadu, India
6 Department of Electrical and Electronics Engineering, Panimalar Engineering College, Tamil Nadu, India
7 Department of Electrical and Electronics Engineering, Karunya Institute of Technology & Sciences, Tamil Nadu, India
* Corresponding Author: Nisha Vasudevan. Email:
Energy Engineering 2023, 120(8), 1747-1761. https://doi.org/10.32604/ee.2023.027821
Received 16 November 2022; Accepted 13 February 2023; Issue published 05 June 2023
Abstract
MigroGrid (MG) has emerged to resolve the growing demand for energy. But because of its inconsistent output, it can result in various power quality (PQ) issues. PQ is a problem that is becoming more and more important for the reliability of power systems that use renewable energy sources. Similarly, the employment of nonlinear loads will introduce harmonics into the system and, as a result, cause distortions in the current and voltage waveforms as well as low power quality issues in the supply system. Thus, this research focuses on power quality enhancement in the MG using hybrid shunt filters. However, the performance of the filter mainly depends upon the design, and stability of the controller. The efficiency of the proposed filter is enhanced by incorporating an enhanced adaptive fuzzy neural network (AFNN) controller. The performance of the proposed topology is examined in a MATLAB/Simulink environment, and experimental findings are provided to validate the effectiveness of this approach. Further, the results of the proposed controller are compared with Adaptive Fuzzy Back-Stepping (AFBS) and Adaptive Fuzzy Sliding (AFS) to prove its superiority over power quality improvement in MG. From the analysis, it can be observed that the proposed system reduces the total harmonic distortion by about 1.8%, which is less than the acceptable limit standard.Keywords
Microgrids are power systems that can provide electricity by combining a number of local loads with distributed generation resources, which can be evolved to address the rising demand for electric power. However, due to weather factors including variable irradiation, wind speed, and the variation of the load applied to the grid, major power quality (PQ) issues such as sag, etc. [1]. Hence, to overcome this, various topologies have emerged to mitigate the PQ issues in MG.
A revolutionary electric utility method is proposed for smart grid consumers because significant retailers offer various time-varying pricing structures. Thus, to investigate the characteristics and behaviour of the batch Q training method, comprehensive evaluations and comparisons are undertaken using a statistical approach. With the implementation of the CFS technique and the KNN learning algorithm, cyber attack identification for SCADA power distribution has been developed. To increase the detection rate of SCADA, cyberattacks used the features extracted from power measurements [2].
Effective monitoring and control of DG improve the efficiency of SG. Image processing and machine learning techniques are commonly used to determine turbine engine speed. To become more precise, a video feed is continuously acquired from integrated camera sensors fixed on the wind energy tower. The video is then sent to a central server, which is an elevated receiver capable of performing sophisticated algorithms and estimating the turbine speed With an accuracy about 96% [3].
A randomised response based on a SG data aggregation approach that satisfies local differential privacy (LDP) has been formulated. This method can estimate the supply and demand of SG effectively and protects the privacy of each participant. This system is more effective for SG [4].
Voltage regulation plays a major role in the electric grid. This can be enhanced by implementing an On-load tap Changer (OLTP) on the primary substation transformer, but the implementation on OLTP improves only the reactive power compensation only not all the power quality issues [5].
Reference [6] described a hierarchical automatic categorization technique for ED events that divides them into 3 categories: legitimate data, switch activities, and faults or power quality disturbances. 92.9% of the 140 COMTRADE files that were examined by this process were appropriately classified into one of these groups. This work also provides an automated method for classifying magnetic inrush incidents in addition to this categorization. Using 32 transformer inrush COMTRADE data, a classification rate of 90.63% is shown.
Using a cyber-security study and HIL modelling, the characterization of power networks and their hazards have been analysed. The physical protection measure for power grid and its computation are carried out by utilizing Markovian Methodology, and co-simulation is performed represent the grid’s overall security [7].
Despite the many advantages of DG, such as environmental friendliness and so forth, there are still a number of difficulties associated with integrating DG with the grid. The power quality issues in DG can be eliminated using UPQC. Hence, by implementing a modified version of the synchronous reference frame (SRF), a novel UPQC is formulated [8].
The real characteristics of the existing distribution network and its elements (e.g., storage devices) are mostly ignored. Such approximations may be tolerable in the event of a massive system, but they may create incredible outcomes. It is essentially uncertain to describe the operating policy of SG with demand-side technologies when they are integrated into power market operations [9–11]. By regulating the network communication allocation and signal strength, a D2D radio resource management strategy is incorporated into SG to associate all energy management units together.
Energy management in industry plays a vital role in framework of energy. Hence, a study of individual industrial consumer demand for energy along with a financial load model is carried out. This work modelled a programme to lower utility loads during peak load condition and offers an alluring incentive for industrial consumers [12].
A predictive control-based solution to combined PEV recharging planning and power regulation is being developed with the intention of reducing either PEV recharging or electric generation costs [13].
DG systems such as PV, wind, and fuel cell-powered RES are modelled for standalone operations. To enhance the efficacy of the DG system, a SRF and MSRF powered UPQC is designed. The suggested UPQC model was analysed for its ability to mitigate voltage instability and compensate distortions irrespective of supply voltage conditions [14].
In light of a virtual SOC model and exploiting current, a multi-objective power management methodology for an MMC-based EV armada was incorporated into the savvy network. The MMC-based strategy controls the charging and discharging and interactive power management between EVs and utilities. It also thereby reduces the adverse effects of large-scale application of EVs on the utility grid [15,16].
Recently, a new danger known as cyber-physical attacks targeted the power grid operations. A novel approach can be developed successfully and repeatedly so as to trick the control centre into identifying false line outage positions [17,18].
Most historical research focuses on reactive power management or harmonic network disturbances. The suggested CPD is an upgraded APLC architecture that can regulate the voltage stability of each bus, and the whole system is performing reactive power compensation by inserting appropriate corrective active and reactive current flow during both fundamental and harmonic frequencies [19].
From quantitative viewpoints, the authors [20] analysed the smart grid’s data transmission, including bit rates, dependability, safety and adaptability, using NB-IoT. Through Monte Carlo simulations, the performance of NB-IoT in SG communication contexts, such as urban and rural areas is assessed.
A nonlinear grid model with a focus on the false data attacks (FDAs) in SG is developed. A blind and non-blind FDA criteria are derived to secure SG from the false attacks. A focused blind FDA may change the intended state with further knowledge of the hijacked system states [21].
A unique reinforcement-learning-based energy control and management strategy for SG is developed. To deliver the best power dispatch and reduce overall operating costs, a unique stochastic framework is developed. The suggested approach makes use of Q-learning technology to anticipate the output power of the wind and PV. An optimisation strategy using dragonflies is developed due to its enormous complexity and nonlinearity [22].
Technology covers a variety of new methods for increasing customer participation and improving network functioning. The implementation of innovative load forecasting and the smart metre has provided unprecedented flexibility to the electricity market. Other technical advancements, such as PV, storage systems, electric automobiles, and communication applications, have enabled cost-saving and innovative service delivery strategies to meet the power demand [23].
The authors of [24] suggested a polymorphic heterogeneity edge-based distributed generation security infrastructure. PHSA combines a closed-loop feedback process due to the dynamic hierarchical redundancies structure to overcome equipment and software similarities, singleness, and immobility problems in the SG infrastructure. Furthermore, security and serviceability assessment, multi-state provider reaction smart arbitration, and security risk motion are presented to formulate the closed-loop core internal controller.
Unlike large central power stations, PV DG units may be located at or near the load. So PSO is incorporated for optimum PV distribution. With this MPPT condition, an objective function is introduced that incorporates technological and financial views of optimising renewable power utilisation [25–28].
Even though numerous controllers have been developed for SAF, they are limited by some constraints. In order to compensate for the harmonic and reactive power in MG, the controller has to produce appropriate compensation signals for the filter. Thus, this work proposes an AFNN topology for control purposes. Due to its stable nature, AFNN is expected to enhance the power quality of MG. It also enhances dynamic power performances such as reduced THD, etc. The NTSMBS technique is proposed for the existing controller to overcome singularity issues and reach a quick resolution. To lessen the necessity for an extensive network system for SHF due to the impact of unexpected perturbations due to load variations, an AFNN by the suggested NTSMC is employed. The primary goal of this work is to assess the harmonic disruptions in the SG, propose an efficient way to keep harmonic components within IEEE-519 requirements, and build an adaptive approach using NTSMC to provide high accuracy and discrete regulation for SHF. The suggested method’s evaluation is performed using two distinct types of controllers: adaptive fuzzy sliding control and adaptive fuzzy back-stepping control.Their performance analysis is carried out on the MATLAB platform. As a result, the focus of this study is the development of an improved control method, the AFNN controller employing NTSMC, for regulating and producing PWM signals for SHF VSI. The structure of the article is as follows: The system architecture is explained in Part 2, the control strategies of SHF are covered in Part 3, the data and analysis are presented in Part 4, and the conclusion is in Part 5.
Fig. 1a depicts the relationship between PQ, sustainable source, and SG. This power triangle relationship is essential to enhancing the PQ, which will become even more critical in the upcoming days. SG denotes contemporary voltage stability in motion. Incorporating renewable energy sources into the power grid necessitates a structural shift in the utilisation of renewable energy resources. Fig. 1b depicts the structure of SG.
Figure 1: (a) The structural outline depicts the link between the smart grid, sustainable technologies, and power quality. (b) A typical smart grid architecture
Fig. 2 depicts the system of a 3-phase filter element coupled with the MG. The main focus of this study is to design novel regulating filters using intelligent techniques that enhance the power quality in the smart grid structure. The arrangement of proposed MG comprises a source (which includes a solar, wind, and battery), an SHF, DC-DC power converters, and a load. Vs1, Vs2 and Vs3 denote grid voltage and Is1, Is2, and Is3 denote the supply currents. The voltage at the intersection point is denoted by V1, V2, and V3, while IL1, IL2 and IL3 denote the load currents. C and Vdc refer the capacitance’s and DC bus voltages. Lc and Rc are the ac side inductor and resistor.
Figure 2: Proposed system design
3 Various Approaches in Control Techniques
The SHF proposes a novel management approach for improving power quality in MG and SG. As a result, the proposed approach employs an AFNN-based controller to provide better control and overcome the restrictions associated with the traditional controller. Figs. 3 and 4 show the setup and schematic diagram of an AFNN employing NTSMC. The AFNN system’s inputs are tracking errors, and its outputs are controlled effort vectors. Because it is a four-layer network, the serial connection of each level in AFNN is deduced as follows:
Figure 3: Arrangement of a 4-level Adaptive Fuzzy-Neural-Network
Figure 4: Schematic of Adaptive Fuzzy-Neural-Network
4 Findings and Discussion of the Suggested System
The MATLAB/Simulink software is often used to assess the performance of SHF in a grid-connected system. The performance of SHF is verified under different operating circumstances.
4.1 Steady-State Circumstances with Specific RL Load Case
The reliability of the proposed filter is validated with and without the filter under RL load. While observing the system’s performance with filters, the proposed work incorporated three different filter configurations, namely AFS, AFBS, and ANFNN controller-based filters.
Case A: Without filter
Initially, the performance of the MG is analyzed in the absence of a filter. The waveforms of the input voltage, load current, and overall harmonic distortion values are depicted in Figs. 5a and 5b.
Figure 5: (a) Voltage and current characteristics on the supply side without filtering in a resistive inductive load; (b) Total harmonic distortion evaluation of the current at the input without a filter in a resistive inductive load
From Fig. 5b, it is found that the THD is about 32.77%, which is beyond the limitation of the standard condition. Thus, to lower the THD level of the MG, an AFS controller has been incorporated.
Case B: With AFS controller
Fig. 6 depicts the waveform of the input voltage, load current, and overall THD of the MG with the AFS controller.
Figure 6: (a) Adaptive fuzzy sliding controllers’ efficiency in resistive inductive loads, displaying supply current, filtering current, and capacitor voltage; (b) Total harmonic distortion evaluation of source current utilising adaptive fuzzy sliding in resistive inductive loads
From Fig. 6, it can be observed that the current is about 18 amps and the Vdc attains the maximum DC voltage of 750 V at 0.12 s. The overshoot in this condition is approximately 8.08%. Fig. 6b depicts the THD of the source current, which is about 3.42%.
Case C: With AFBS controller
Fig. 7 portrays the performance evaluation of the MG with the proposed AFBS controller. The DC link voltage of about 760 V is reached at 0.132 s. The overshoot and THD are about 7.22% and 2.24%, respectively.
Figure 7: (a) Adaptive fuzzy back stepping controller’s efficiency in resistive inductive load, displaying supply current, filtering current, and capacitor voltage; (b) Total harmonic distortion evaluation of source current utilising adaptive fuzzy back stepping in resistive inductive load
Case D: With AFNN controller
Thus, the performance evaluation of the SHF filter with the AFNN controller is depicted in Fig. 8. While implementing the AFNN controller, a maximum DC bus voltage of 760 V is achieved with a settling time of 0.06 s, as shown in Fig. 8a. The proportion of overshoot is about 5.33% and exhibits 1.83% of THD. Thus, the overall performance of the MG with and without SHF is illustrated in Table 1.
Figure 8: (a) Adaptive Fuzzy-Neural-Network controller’s efficiency in resistive inductive load, displaying supply current, filtering current, and capacitor voltage; (b) Total harmonic distortion evaluation of source current utilising Adaptive Fuzzy-Neural-Network in resistive inductive load
From the table, it can be seen that among the proposed controllers, AFNN with SHF achieves lower THD when compared to the other two, AFBS and AFS. Hence, it is concluded that AFNN with SHF is the most suitable controller for MG.
4.2 Overall Analysis of the Scheme in Varying Load Situations
In this section, the performance analysis of the different controllers under different load scenarios is analysed. Under AFS control, the load applied to the system is doubled as the rated load at 0.3 s. As a result, there will be a variation in source and load current, as depicted in Fig. 9. After 0.6 s, again, the load remains to increase, and the characteristic acts appropriately. The settling time of the DC bus voltage is 0.45 s, and the peak overshoot is nearly 5.22%.
Figure 9: Response of the system with the AFS controller under load-varying conditions
Fig. 9 depicts the waveform of the supply voltage, supply and load current, filter current, and DC voltage with the AFS controller. Figs. 10 and 11 show the performance of the system with AFBS and AFNN controllers. According to these figures, the load and source current values their maximum value when the load is doubled. From the figures, it is observed that under the AFBS controller, the settling time, maximum value and peak overshoot is about 0.46 s, 768 V and 5.08%. Similarly for the AFNN controller, they are around 0.38 s, 786 V, and 4.68%.
Figure 10: Response of the system with the AFBS controller under load-varying conditions
Figure 11: Response of the system with the AFNN controller under load-varying conditions
4.3 Performance of the System during Imbalanced Non-Linear RL-Loading
The performance of the proposed controllers is also verified under an imbalanced non-linear dynamic load. Fig. 12 depicts the performance of the MG without a filter. Under this condition, the RMS values of 3-phase load currents are 20.08, 16.92, and 23.30 A, respectively. Figs. 12b–12d show the THD analysis for specific load currents. It is about 16.59%, 18.69%, and 13.54% for A, B, and C, respectively.
Figure 12: (a) 3 phase supply voltage and current at load during disturbed resistive inductive loads, (b) THD evaluation for Phase ‘A’ (c) THD evaluation for Phase ‘B’ and (d) THD evaluation for Phase ‘C’
Figs. 13–15 depict the simulation results of the MG under an unbalanced condition with different controllers. Table 2 displays the RMS values of input current utilising various control methods.
Figure 13: (a) Overall system analysis with an AFS controller during disturbed resistive inductive loads, displaying 3 phase supply currents, current at the filtering side, and DC bus voltage. (b) Supply current harmonic evaluation
Figure 14: (a) Overall system analysis with an AFBS controller during disturbed resistive inductive loads, displaying 3 phase supply currents, current at the filtering side, and DC bus voltage. (b) Supply current harmonic evaluation
Figure 15: (a) Overall analysis of the system with an AFNN controller during disturbed resistive inductive loads, displaying 3 phase supply currents, current at the filtering side, and DC bus voltage. (b) Supply current harmonic evaluation
While implementing AFS, AFBS, and AFNN controllers, the ripples at DC bus voltage are about 0.85%, 1.08%, and 0.58%, respectively. From the table, it is apparent that SHF with AFNN exhibits lower THD compared to other controllers.
4.4 The System’s Performance as Wind Speed and Sun Irradiation Fluctuate
The efficiency of the proposed SHF under varying natures of solar PV irradiation and wind velocity is evaluated in this section with the battery’s state of charge equal to 100%. The parameters of the load voltage, input current, DC bus voltage, SOC% of the battery, power from solar sources (PPV), power from wind sources (PWT), and storage device power are depicted in Fig. 16 (Pbatt). Fig. 16 shows the performance of the MG incorporating SHF under variations in irradiation and wind speed.
Figure 16: Performances of the MG incorporating SHF as solar irradiance and wind speed variation
The performance evaluation of this system is verified using dSPACE using the AFNN controller, which exhibits superiority over other controllers. The experimental investigation for harmonic mitigation is carried out under steady-state conditions with RL load and is shown in Fig. 17a. The proposed experimental set-up comprises a digital storage oscilloscope, digital processing boards (DS1208), and an AD converter. Furthermore, Hall effect detectors are incorporated to measure supply currents and voltages. Thus, the AFNN with NTSMC is developed in Simulink and transferred to dSPACE to generate switching pulses for the SHF for harmonic correction. The findings of the experimental verification are shown in Fig. 17b.
Figure 17: AFNN experimental study under steady-state settings (a) Experiment setup using dSPACE, (b) RL load
From the analysis of both simulation and experimental analysis, it is found that AFNN exhibits superior performance than the other two controllers.
The integration of RES sources with the MG causes more power quality issues. As a result, this research incorporated an enhanced SHF in conjunction with SG to diminish the power quality issues of SG. The proposed work formulated three controllers (AFS, AFBS, and AFNN) for SHF, and their efficiencies were examined using MATLAB simulation. The suggested scheme performs well in steady-state, dynamic loading situations, and imbalanced non-linear loading variations. The simulated outcomes under various operating situations show that the suggested technique delivers superior efficiency, stability, and outstanding robustness under different operating conditions. While comparing the performance of the three controllers, AFNN outperforms other controllers in terms of THD reduction, which is about 1.830% under steady-state conditions and similarly under unbalanced conditions as well. The suggested controller’s evaluation is performed once again in an MG scenario with varying sun irradiance and wind velocity. From both the simulation and experimental results, it can be found that AFNN-based SHF provides a better solution to the power quality issues of SG.
Funding Statement: The authors received no specific funding for this study.
Conflicts of Interest: The authors declare that they have no conflicts of interest to report regarding the present study.
References
1. Lu, T., Chen, X., McElroy, M. B., Nielsen, C. P., Wu, Q. et al. (2021). A reinforcement learning-based decision system for electricity pricing plan selection by smart grid end users. IEEE Transactions on Smart Grid, 12(3), 2176–2187. [Google Scholar]
2. Gumaei, A., Hassan, M. M., Huda, S., Hassan, M. R., Camacho, D. et al. (2020). A robust cyberattack detection approach using optimal features of SCADA power systems in smart grids. Applied Soft Computing, 96, 106658. [Google Scholar]
3. Bahaghighat, M., Abedini, F., Xin, Q., Zanjireh, M. M., Mirjalili, S. (2021). Using machine learning and computer vision to estimate the angular velocity of wind turbines in smart grids remotely. Energy Reports, 7, 8561–8576. [Google Scholar]
4. Gai, N., Xue, K., He, P., Zhu, B., Liu, J. et al. (2020). An efficient data aggregation scheme with local differential privacy in smart grid. 2020 16th International Conference on Mobility, Sensing and Networking, pp. 73–80. Tokyo, Japan, IEEE. [Google Scholar]
5. Giacomuzzi, S., Langwasser, M., De Carne, G., Buja, G., Liserre, M. (2021). Smart transformer-based medium voltage grid support by means of active power control. China Electrotechnical Society Transactions on Electrical Machines and Systems, 4(4), 285–294. [Google Scholar]
6. Wilson, J., Reising, D. R., Hay, R. W., Johnson, R. C., Karrar, A. A. et al. (2020). Automated identification of electrical disturbance waveforms within an operational smart power grid. IEEE Transactions on Smart Grid, 11(5), 4380–4389. [Google Scholar]
7. Akaber, P., Moussa, B., Ghafouri, M., Atallah, R., Agba, B. L. et al. (2019). CASeS: Concurrent contingency analysis-based security metric deployment for the smart grid. IEEE Transactions on Smart Grid, 11(3), 2676–2687. [Google Scholar]
8. Lee, K., Liu, H., Fuhs, D., Kores, A., Waffenschmidt, E. (2020). Smart lighting systems as a demand response solution for future smart grids. IEEE Journal of Emerging and Selected Topics in Power Electronics, 8(3), 2362–2370. [Google Scholar]
9. Guan, A., Guan, D. J. (2020). An efficient and privacy protection communication scheme for smart grid. IEEE Access, 8, 179047–179054. [Google Scholar]
10. Kong, P. Y., Song, Y. (2020). Joint consideration of communication network and power grid topology for communications in community smart grid. IEEE Transactions on Industrial Informatics, 16(5), 2895–2905. [Google Scholar]
11. Haghighat, H., Karimianfard, H., Zeng, B. (2020). Integrating energy management of autonomous smart grids in electricity market operation. IEEE Transactions on Smart Grid, 11(5), 4044–4055. [Google Scholar]
12. Bagdadee, H., Aurangzeb, M., Ali, S., Zhang, L. (2020). Energy management for the industrial sector in smart grid system. Energy Reports, 6, 1432–1442. [Google Scholar]
13. Vincent Poor, H., Shi, Y., Tuan, H. D., Savkin, A. V., Duong, T. Q. (2019). Model predictive control for smart grids with multiple electric-vehicle charging stations. IEEE Transactions on Smart Grid, 10(2), 2127–2136. [Google Scholar]
14. Samal, S., Hota, P. K., Barik, P. K. (2020). Performance improvement of a distributed generation system using unified power quality conditioner. Technology and Economics of Smart Grids and Sustainable Energy, 5, 24. [Google Scholar]
15. Gope, P., Sikdar, B. (2019). Privacy-aware authenticated key agreement scheme for secure smart grid communication. IEEE Transactions on Smart Grid, 10(4), 3953–3962. [Google Scholar]
16. Mao, M., Ding, Y., Chang, L., Hatziargyriou, N. D., Chen, Q. et al. (2019). Multi-objective power management for EV fleet with MMC-based integration into smart grid. IEEE Transactions on Smart Grid, 10(2), 1428–1439. [Google Scholar]
17. Shum, C., Lau, W. H., Mao, T., Chung, H. S. H., Tsang, K. F. et al. (2018). Co-simulation of distributed smart grid software using direct-executionsimulation. IEEE Access, 6, 20531–20544. [Google Scholar]
18. Chung, H. M., Li, W. T., Yuen, C., Chung, W. H., Zhang, Y. et al. (2019). Local cyber-physical attack for masking line outage and topology attack in smart grid. IEEE Transactions on Smart Grid, 10(4), 4577–4588. [Google Scholar]
19. Moghbel, M., Masoum, M. A. S., Fereidouni, A., Deilami, S. (2018). Optimal sizing, siting and operation of custom power devices with STATCOM and APLC functions for real-time reactive power and network voltage quality control of smart grid. IEEE Transactions on Smart Grid, 9(6), 5564–5575. [Google Scholar]
20. Li, Y., Cheng, X., Cao, Y., Wang, D., Yang, L. (2018). Smart choice for the smart grid: Narrowband Internet of Things (NB-IoT). IEEE Internet of Things Journal, 5(3), 1505–1515. [Google Scholar]
21. Chin, W. L., Lee, C. H., Jiang, T. (2018). Blind false data attacks against AC state estimation based on geometric approach in smart grid communications. IEEE Transactions on Smart Grid, 9(6), 6298–6306. [Google Scholar]
22. Wei, L., Yi, C., Yun, J. (2021). Energy drive and management of smart grids with high penetration of renewable sources of wind unit and solar panel. International Journal of Electrical Power & Energy Systems, 129, 106846. [Google Scholar]
23. Sadjadi, E. N. (2020). Service dominant logic of marketing in smart grids. The Electricity Journal, 33(7), 106797. [Google Scholar]
24. Wang, Z., Jiang, D., Wang, F., Lv, Z., Nowak, R. (2021). A polymorphic heterogeneous security architecture for edge-enabled smart grids. Sustainable Cities and Society, 67, 102661. [Google Scholar]
25. Radoglou-Grammatikis, P., Sarigiannidis, P., Iturbe, E., Rios, E., Martinez, S. et al. (2021). SPEAR SIEM: A security information and event management system for the smart grid. Computer Networks, 193, 108008. [Google Scholar]
26. Eltamaly, A. M., Mohamed, Y. S., El-Sayed, A. H. M., Mohamed, M. A., Elghaffar, A. N. A. (2020). Power quality and reliability considerations of photovoltaic distributed generation. Technology and Economics of Smart Grids and Sustainable Energy, 5(1), 1–21. [Google Scholar]
27. Cholamuthu, P., Irusappan, B., Paramasivam, S. K., Ramu, S. K., Muthusamy, S. et al. (2022). A grid-connected solar PV/Wind turbine based hybrid energy system using ANFIS controller for hybrid series active power filter to improve the power quality. International Transactions on Electrical Energy Systems, 2022, 1–14. [Google Scholar]
28. Ananthi, K., Manoharan, S. (2022). Enhancing power quality using packed U cell 5 multilevel inverter-based shunt active power filter with fuzzy controller. International Journal of Fuzzy Systems, 24, 1356–1370. [Google Scholar]
Cite This Article
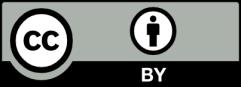