Open Access
ARTICLE
A Framework for Driver Drowsiness Monitoring Using a Convolutional Neural Network and the Internet of Things
1 School of Computing, Telkom University, Bandung, 40257, Indonesia
2 Faculty of Information Science and Technology, Universiti Kebangsaan Malaysia, Bangi, 43600, Malaysia
3 Department of Electrical Engineering, College of Engineering, Qassim University, Al-Gassim, 51411, Saudi Arabia
4 Graduated School of Business, Universiti Kebangsaan Malaysia, Bangi, 43600, Malaysia
* Corresponding Author: Muhamad Irsan. Email:
Intelligent Automation & Soft Computing 2024, 39(2), 157-174. https://doi.org/10.32604/iasc.2024.042193
Received 22 May 2023; Accepted 16 January 2024; Issue published 21 May 2024
Abstract
One of the major causes of road accidents is sleepy drivers. Such accidents typically result in fatalities and financial losses and disadvantage other road users. Numerous studies have been conducted to identify the driver’s sleepiness and integrate it into a warning system. Most studies have examined how the mouth and eyelids move. However, this limits the system’s ability to identify drowsiness traits. Therefore, this study designed an Accident Detection Framework (RPK) that could be used to reduce road accidents due to sleepiness and detect the location of accidents. The drowsiness detection model used three facial parameters: Yawning, closed eyes (blinking), and an upright head position. This model used a Convolutional Neural Network (CNN) consisting of two phases. The initial phase involves video processing and facial landmark coordinate detection. The second phase involves developing the extraction of frame-based features using normalization methods. All these phases used OpenCV and TensorFlow. The dataset contained 5017 images with 874 open eyes images, 850 closed eyes images, 723 open-mouth images, 725 closed-mouth images, 761 sleepy-head images, and 1084 non-sleepy head images. The dataset of 5017 images was divided into the training set with 4505 images and the testing set with 512 images, with a ratio of 90:10. The results showed that the RPK design could detect sleepiness by using deep learning techniques with high accuracy on all three parameters; namely 98% for eye blinking, 96% for mouth yawning, and 97% for head movement. Overall, the test results have provided an overview of how the developed RPK prototype can accurately identify drowsy drivers. These findings will have a significant impact on the improvement of road users’ safety and mobility.Keywords
Cite This Article
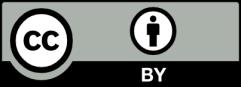