Open Access
ARTICLE
Case Optimization Using Improved Genetic Algorithm for Industrial Fuzzing Test
1 School of Information, Liaoning University, Shenyang 110036, China
2 School of Physics, Liaoning University, Shenyang 110036, China
3 School of Computer Science & Cyperspace Security, Hainan University, Haikou 570228, China
4 Faculty of Arts, The University of British Columbia, Vancouver, BC V6T 1Z4, Canada
* Corresponding Author: Jiangyuan Yao. Email:
Intelligent Automation & Soft Computing 2021, 28(3), 857-871. https://doi.org/10.32604/iasc.2021.017214
Received 24 January 2021; Accepted 27 February 2021; Issue published 20 April 2021
Abstract
Due to the lack of security consideration in the original design of industrial communication protocols, industrial fuzzing test which can successfully exploit various potential security vulnerabilities has become one new research hotspot. However, one critical issue is how to improve its testing efficiency. From this point of view, this paper proposes a novel fuzzing test case optimization approach based on improved genetic algorithm for industrial communication protocols. Moreover, a new individual selection strategy is designed as the selection operator in this genetic algorithm, which can be actively engaged in the fuzzing test case optimization process. In this individual selection strategy, the selection operation based on high and low fitness populations is introduced to enhance the individual selection diversity, which can increase the average fitness value of individuals and further improve the efficiency of test cases. In practice, we construct industrial communication data which conforms to Siemens S7 communication protocol to evaluate the proposed approach, and the experimental results show that, the individual fitness value of output population in the improved genetic algorithm is obviously higher than the one in traditional genetic algorithm under the same iteration, and this approach can enhance the efficiency and accuracy of test cases in Siemens S7 fuzzing vulnerability exploiting.Keywords
Cite This Article
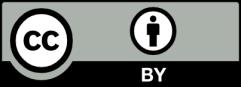