Open Access
ARTICLE
Intrusion Detection Using a New Hybrid Feature Selection Model
Computer Science Department, The World Islamic Sciences and Education University, Amman, 1101-11947, Jordan
* Corresponding Author: Adel Hamdan Mohammad. Email:
Intelligent Automation & Soft Computing 2021, 30(1), 65-80. https://doi.org/10.32604/iasc.2021.016140
Received 24 December 2020; Accepted 13 April 2021; Issue published 26 July 2021
Abstract
Intrusion detection is an important topic that aims at protecting computer systems. Besides, feature selection is crucial for increasing the performance of intrusion detection. This paper employs a new hybrid feature selection model for intrusion detection. The implemented model uses Grey Wolf Optimization (GWO) and Particle Swarm Optimization (PSO) algorithms in a new manner. In addition, this study introduces two new models called (PSO-GWO-NB) and (PSO-GWO-ANN) for feature selection and intrusion detection. PSO and GWO show emergent results in feature selection for several purposes and applications. This paper uses PSO and GWO to select features for the intrusion detection system. Furthermore, in this study, a new emergent feature selection method using the interstation of (PSO and GWO) features is developed. Also, this research examines the Most frequently Repeated Features from (PSO and GWO) and gives it the name (MRF). This study runs PSO and GWO for a specific number of iterations, which the user could define. Each feature selection model runs independently, and the selected feature set is saved. PSO features, GWO features, the intersection of (PSO and GWO) features, and MRF features are tested at the next stage. This research uses the UNSW-NB15 dataset for evaluation purposes. Furthermore, experiments are implemented using two classifiers: Naïve Bayesian (NB) and Artificial Neural Networks (ANN). The results show that PSO and GWO are highly acceptable for the selection of intrusion detection features. Besides, the intersection of (PSO and GWO) features gives an emergent result with a minimum number of features. Moreover, MRF features show highly acceptable results. The evaluation process criteria are true positive, false positive, false negative, precision, and recall. The experiments demonstrate that MRF features give a good result related to precision and recall. Finally, experiments show that the performance of (PSO-GWO-NB) classifier is better than (PSO-GWO-ANN) for feature selection and intrusion detections.Keywords
Cite This Article
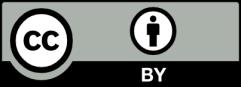