Open Access
ARTICLE
An Intelligent Business Model for Product Price Prediction Using Machine Learning Approach
1 Department of Software Engineering, Mehran University of Engineering & Technology, Jamshoro, 76062, Pakistan
2 College of Computer Science and Information Systems, Najran University, Najran, 61441, Saudi Arabia
3 College of Computer Science and Engineering, University of South Florida, Tampa, 33620, USA
* Corresponding Author: Asadullah Shaikh. Email:
Intelligent Automation & Soft Computing 2021, 30(1), 147-159. https://doi.org/10.32604/iasc.2021.018944
Received 27 March 2021; Accepted 28 April 2021; Issue published 26 July 2021
Abstract
The price of a product plays a vital role in its market share. Customers usually buy a product when it fits their needs and budget. Therefore, it is an essential area in the business to make decisions about prices for each product. The major portion of the business profit is directly connected with the percentage of the sale, which relies on certain factors of customers including customers’ behavior and market competitors. It has been observed in the past that machine learning algorithms have made the decision-making process more effective and profitable in businesses. The fusion of machine learning with business intelligence systems has produced potentially better results for business workers. This study proposes a fusion model of machine learning and business intelligence to facilitate the effective pricing mechanism of products. Three machine learning algorithms namely (1) Multiclass Random Forest, (2) Multiclass Logistic Regression and (3) Multiclass one-vs-all have been applied for product price prediction. This intelligent business model helps business workers to set product pricing and discounts based on customer’s buying behavior (i.e., customer’s average amount spent in buying items). The model also predicts the demand for the product in the market, which may help in making effective decisions about the product to gain a better productivity. The performance of the model has been evaluated using hold-out cross validation. Multiclass one-vs-all out performed the other two algorithms in terms of average log-loss and it produced better accuracy in prediction for both hold-out as well as cross validation. The outcomes of the proposed model may help businesses in exploring business data and to gain higher share in the market.Keywords
Cite This Article
Citations
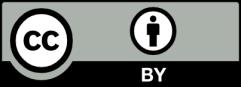