Open Access
ARTICLE
Kidney Tumor Segmentation Using Two-Stage Bottleneck Block Architecture
1 Department of Computer Engineering, Kirikkale University, Kirikkale, 71450, Turkey
2 Department of Electrical & Electronics Engineering, Kirikkale University, Kirikkale, 71450, Turkey
3 Department of Computer Engineering, Faculty of Technology, Gazi University, Ankara, 06560, Turkey
4 Department of Electrical & Electronics Engineering, Kirikkale University, Kirikkale, 71450, Turkey
* Corresponding Author: Fuat Turk. Email:
Intelligent Automation & Soft Computing 2022, 33(1), 349-363. https://doi.org/10.32604/iasc.2022.023710
Received 18 September 2021; Accepted 03 November 2021; Issue published 05 January 2022
Abstract
Cases of kidney cancer have shown a rapid increase in recent years. Advanced technology has allowed bettering the existing treatment methods. Research on the subject is still continuing. Medical segmentation is also of increasing importance. In particular, deep learning-based studies are of great importance for accurate segmentation. Tumor detection is a relatively difficult procedure for soft tissue organs such as kidneys and the prostate. Kidney tumors, specifically, are a type of cancer with a higher incidence in older people. As age progresses, the importance of having diagnostic tests increases. In some cases, patients with kidney tumors may not show any serious symptoms until the last stage. Therefore, early diagnosis of the tumor is important. This study aimed to develop support systems that could help physicians in the segmentation of kidney tumors. In the study, improvements were made on the encoder and decoder phases of the V-Net model. With the double-stage bottleneck block structure, the architecture was transformed into a unique one, which achieved an 86.9% kidney tumor Dice similarity coefficient. The results show that the model gives applicable and accurate results for kidney tumor segmentation.Keywords
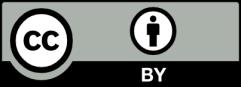