Open Access
ARTICLE
A Hybrid Deep Features PSO-ReliefF Based Classification of Brain Tumor
Department of Radiology, College of Medicine, Qassim University, Buraidah, 52571, Saudi Arabia
* Corresponding Author: Alaa Khalid Alduraibi. Email:
Intelligent Automation & Soft Computing 2022, 34(2), 1295-1309. https://doi.org/10.32604/iasc.2022.026601
Received 30 December 2021; Accepted 31 January 2022; Issue published 03 May 2022
Abstract
With technological advancements, deep machine learning can assist doctors in identifying the brain mass or tumor using magnetic resonance imaging (MRI). This work extracts the deep features from 18-pre-trained convolutional neural networks (CNNs) to train the classical classifiers to categorize the brain MRI images. As a result, DenseNet-201, EfficientNet-b0, and DarkNet-53 deep features trained support vector machine (SVM) model shows the best accuracy. Furthermore, the ReliefF method is applied to extract the best features. Then, the fitness function is defined to select the number of nearest neighbors of ReliefF algorithm and feature vector size. Finally, the particle swarm optimization algorithm minimizes the fitness function to determine the optimal feature vector for model training. The proposed approach is validated by using the available online dataset. The proposed approach enhances the classification accuracy to 97.1% using the optimal concatenated deep features of DenseNet-201and DarkNet-53. Therefore, owing to the high accuracy of the proposed approach, it can be helpful to use real-time applications in the future.Keywords
Cite This Article
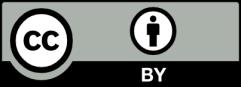