Open Access
ARTICLE
Application of CNN and Long Short-Term Memory Network in Water Quality Predicting
1 College of Engineering and Design, Hunan Normal University, Changsha, 410081, China
2 Hunan Institute of Metrology and Test, Changsha, 410014, China
3 Big Data Institute, Hunan University of Finance and Economics, Changsha, 410205, China
4 University Malaysia Sabah, Sabah, 88400, Malaysia
* Corresponding Author: Jianjun Zhang. Email:
Intelligent Automation & Soft Computing 2022, 34(3), 1943-1958. https://doi.org/10.32604/iasc.2022.029660
Received 09 March 2022; Accepted 19 April 2022; Issue published 25 May 2022
Abstract
Water resources are an indispensable precious resource for human survival and development. Water quality prediction plays a vital role in protecting and enhancing water resources. Changes in water quality are influenced by many factors, both long-term and short-term. Therefore, according to water quality changes’ periodic and nonlinear characteristics, this paper considered dissolved oxygen as the research object and constructed a neural network model combining convolutional neural network (CNN) and long short-term memory network (LSTM) to predict dissolved oxygen index in water quality. Firstly, we preprocessed the water quality data set obtained from the water quality monitoring platform. Secondly, we used a CNN network to extract local features from the preprocessed water quality data and transferred time series with better expressive power than the original water quality information to the LSTM layer for prediction. We choose optimal parameters by setting the number of neurons in the LSTM network and the size and number of convolution kernels in the CNN network. Finally, LSTM and the proposed model were used to evaluate the water quality data. Experiments showed that the proposed model is more accurate than the conventional LSTM in the prediction effect of peak fitting. Compared with the conventional LSTM model, its root mean square error, Pearson correlation coefficient, mean absolute error and mean square error were respectively optimized by 5.99%, 2.80%, 2.24%, and 11.63%.Keywords
Cite This Article
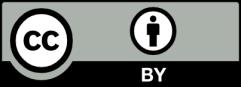