Open Access
ARTICLE
An Optimised Defensive Technique to Recognize Adversarial Iris Images Using Curvelet Transform
1 School of Computing, Department of Networking and Communications, SRM Institute of Science and Technology, Kattankulathur, Chennai, 603203, India
2 School of Computing, Department of Computational Intelligence, SRM Institute of Science and Technology, Kattankulathur, Chennai, 603203, India
* Corresponding Author: K. Meenakshi. Email:
Intelligent Automation & Soft Computing 2023, 35(1), 627-643. https://doi.org/10.32604/iasc.2023.026961
Received 07 January 2022; Accepted 24 February 2022; Issue published 06 June 2022
Abstract
Deep Learning is one of the most popular computer science techniques, with applications in natural language processing, image processing, pattern identification, and various other fields. Despite the success of these deep learning algorithms in multiple scenarios, such as spam detection, malware detection, object detection and tracking, face recognition, and automatic driving, these algorithms and their associated training data are rather vulnerable to numerous security threats. These threats ultimately result in significant performance degradation. Moreover, the supervised based learning models are affected by manipulated data known as adversarial examples, which are images with a particular level of noise that is invisible to humans. Adversarial inputs are introduced to purposefully confuse a neural network, restricting its use in sensitive application areas such as biometrics applications. In this paper, an optimized defending approach is proposed to recognize the adversarial iris examples efficiently. The Curvelet Transform Denoising method is used in this defense strategy, which examines every sub-band of the adversarial images and reproduces the image that has been changed by the attacker. The salient iris features are retrieved from the reconstructed iris image by using a pre-trained Convolutional Neural Network model (VGG 16) followed by Multiclass classification. The classification is performed by using Support Vector Machine (SVM) which uses Particle Swarm Optimization method (PSO-SVM). The proposed system is tested when classifying the adversarial iris images affected by various adversarial attacks such as FGSM, iGSM, and Deepfool methods. An experimental result on benchmark iris dataset, namely IITD, produces excellent outcomes with the highest accuracy of 95.8% on average.Keywords
Cite This Article
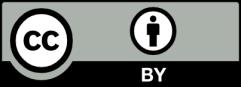