Open Access
ARTICLE
Networking Controller Based Real Time Traffic Prediction in Clustered Vehicular Adhoc Networks
1 Saveetha School of Engineering, Saveetha Institute of Medical and Technical Sciences, Saveetha University, Saveetha Nagar, Thandalam, Chennai, 602105, India
2 Department of Electronics and Communication Engineering, College of Engineering and Technology, SRM Institute of Science and Technology, Vadapalani Campus, Chennai, 600026, India
3 Department of Biomedical Engineering, Saveetha School of Engineering, Saveetha Institute of Medical and Technical Sciences, Saveetha University, Saveetha Nagar, Thandalam, Chennai, 602105, India
* Corresponding Author: S. Srinivasan. Email:
Intelligent Automation & Soft Computing 2023, 35(2), 2189-2203. https://doi.org/10.32604/iasc.2023.028785
Received 17 February 2022; Accepted 14 April 2022; Issue published 19 July 2022
Abstract
The vehicular ad hoc network (VANET) is an emerging network technology that has gained popularity because to its low cost, flexibility, and seamless services. Software defined networking (SDN) technology plays a critical role in network administration in the future generation of VANET with fifth generation (5G) networks. Regardless of the benefits of VANET, energy economy and traffic control are significant architectural challenges. Accurate and real-time traffic flow prediction (TFP) becomes critical for managing traffic effectively in the VANET. SDN controllers are a critical issue in VANET, which has garnered much interest in recent years. With this objective, this study develops the SDNTFP-C technique, a revolutionary SDN controller-based real-time traffic flow forecasting technique for clustered VANETs. The proposed SDNTFP-C technique combines the SDN controller’s scalability, flexibility, and adaptability with deep learning (DL) models. Additionally, a novel arithmetic optimization-based clustering technique (AOCA) is developed to cluster automobiles in a VANET. The TFP procedure is then performed using a hybrid convolutional neural network model with attention-based bidirectional long short-term memory (HCNN-ABLSTM). To optimise the performance of the HCNN-ABLSTM model, the dingo optimization technique was used to tune the hyperparameters (DOA). The experimental results analysis reveals that the suggested method outperforms other current techniques on a variety of evaluation metrics.Keywords
Cite This Article
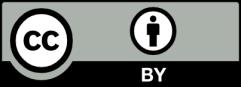