Open Access
ARTICLE
Germination Quality Prognosis: Classifying Spectroscopic Images of the Seed Samples
Department of Information Systems, College of Computer and Information Systems, Umm Al-Qura University, Makkah, Saudi Arabia
* Corresponding Author: Saud S. Alotaibi. Email:
Intelligent Automation & Soft Computing 2023, 35(2), 1815-1829. https://doi.org/10.32604/iasc.2023.029446
Received 03 March 2022; Accepted 15 April 2022; Issue published 19 July 2022
Abstract
One of the most critical objectives of precision farming is to assess the germination quality of seeds. Modern models contribute to this field primarily through the use of artificial intelligence techniques such as machine learning, which present difficulties in feature extraction and optimization, which are critical factors in predicting accuracy with few false alarms, and another significant difficulty is assessing germination quality. Additionally, the majority of these contributions make use of benchmark classification methods that are either inept or too complex to train with the supplied features. This manuscript addressed these issues by introducing a novel ensemble classification strategy dubbed “Assessing Germination Quality of Seed Samples (AGQSS) by Adaptive Boosting Ensemble Classification” that learns from quantitative phase features as well as universal features in greyscale spectroscopic images. The experimental inquiry illustrates the significance of the proposed model, which outperformed the currently available models when performance analysis was performed.Keywords
Cite This Article
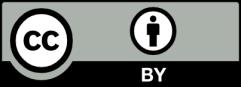